-
-
News
News Highlights
- Books
Featured Books
- smt007 Magazine
Latest Issues
Current IssueWhat's Your Sweet Spot?
Are you in a niche that’s growing or shrinking? Is it time to reassess and refocus? We spotlight companies thriving by redefining or reinforcing their niche. What are their insights?
Moving Forward With Confidence
In this issue, we focus on sales and quoting, workforce training, new IPC leadership in the U.S. and Canada, the effects of tariffs, CFX standards, and much more—all designed to provide perspective as you move through the cloud bank of today's shifting economic market.
Intelligent Test and Inspection
Are you ready to explore the cutting-edge advancements shaping the electronics manufacturing industry? The May 2025 issue of SMT007 Magazine is packed with insights, innovations, and expert perspectives that you won’t want to miss.
- Articles
- Columns
- Links
- Media kit
||| MENU - smt007 Magazine
Managing Big Data from an Analog World
November 18, 2015 | Chandran Nair, National InstrumentsEstimated reading time: 3 minutes
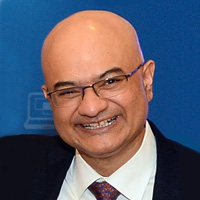
There once was a time when hardware sampling rates, limited by the speed at which analog-to-digital conversion took place, physically restricted how much data was acquired. But the advances in computing technology, including increasing microprocessor speed and hard-drive storage capacity, combined with decreasing costs for hardware and software, have provoked an explosion of data coming in unabated. Among the most interesting to the engineer and scientist is data derived from the physical world. This is analog data that is captured and digitized and otherwise known as “big analog Ddata.” It is collected from measurements of vibration, RF signals, temperature, pressure, sound, image, light, magnetism, voltage, and so on.
In the field of measurement applications, engineers and scientists collect vast amounts of data every minute. For every second that the Large Hadron Collider at the European Organization for Nuclear Research (CERN) runs an experiment, the instrument generates 40TB of data. For every 30 minutes that a Boeing jet engine runs, the system creates 10TB of operations information (Gantz, 2011).
In the age of big data, hardware is evidently no longer the limiting factor in acquisition applications, but the management of acquired data is. How do we store and make sense of data? How do we keep them secured? How do we future proof them? These questions become compounded when systems evolve to become more complex, and the amount of data required to describe those systems grow beyond comprehension. This inevitably results in longer project schedules and less efficiency in development. More advanced tools and smarter measurement systems will be essential to managing this explosion of data and help engineers make informed decisions faster.
For engineers, this means instrumentation must be smarter and sensors, measurement hardware, data buses, and application software need to work together to provide actionable data at the right time. The big data phenomenon adds new challenges to data analysis, search, integration, reporting, and system maintenance that must be met to keep pace with the exponential growth of data. And the sources of data are many. As a result, these challenges unique to big analog data have provoked three technology trends in the widespread field of data acquisition.
Contextual Data Mining
The physical characteristics of some real-world phenomena prevent information from being gleaned unless acquisition rates are high enough, which makes small data sets an impossibility. Even when the characteristics of the measured phenomena allow more information gathering, small data sets often limit the accuracy of conclusions and predictions in the first place.
Consider a gold mine where only 20% of the gold is visible. The remaining 80% is in the dirt where you can’t see it. Mining is required to realize the full value of the contents of the mine. This leads to the term “digital dirt,” meaning digitized data can have concealed value. Hence, data analytics and data mining are required to achieve new insights that have never before been seen.
Data mining is the practice of using the contextual information saved along with data to search through and pare down large data sets into more manageable, applicable volumes. By storing raw data alongside its original context or “metadata,” it becomes easier to accumulate, locate, and later manipulate and understand. For example, examine a series of seemingly random integers: 5126838937. At first glance, it is impossible to make sense of this raw information. However, when given context like (512) 683-8937, the data is much easier to recognize and interpret as a phone number.
Descriptive information about measurement data context provides the same benefits and can detail anything from sensor type, manufacturer, or calibration date for a given measurement channel to revision, designer, or model number for an overall component under test. In fact, the more context that is stored with raw data, the more effectively that data can be traced throughout the design life cycle, searched for or located, and correlated with other measurements in the future by dedicated data post-processing software.
Editor's Note: This article originally appeared in the November 2015 issue of SMT Magazine.
Suggested Items
TRI Unveils New Platform for Diverse Board Sizes
07/14/2025 | TRITest Research, Inc. (TRI), the leading test and inspection systems provider for the electronics manufacturing industry, proudly introduces a new size configuration for SPI and AOI Models.
Specially Developed for Laser Plastic Welding from LPKF
06/25/2025 | LPKFLPKF introduces TherMoPro, a thermographic analysis system specifically developed for laser plastic welding that transforms thermal data into concrete actionable insights. Through automated capture, evaluation, and interpretation of surface temperature patterns immediately after welding, the system provides unprecedented process transparency that correlates with product joining quality and long-term product stability.
Smart Automation: The Power of Data Integration in Electronics Manufacturing
06/24/2025 | Josh Casper -- Column: Smart AutomationAs EMS companies adopt automation, machine data collection and integration are among the biggest challenges. It’s now commonplace for equipment to collect and output vast amounts of data, sometimes more than a manufacturer knows what to do with. While many OEM equipment vendors offer full-line solutions, most EMS companies still take a vendor-agnostic approach, selecting the equipment companies that best serve their needs rather than a single-vendor solution.
Keysight, NTT, and NTT Innovative Devices Achieve 280 Gbps World Record Data Rate with Sub-Terahertz for 6G
06/17/2025 | Keysight TechnologiesKeysight Technologies, Inc. in collaboration with NTT Corporation and NTT Innovative Devices Corporation (NTT Innovative Devices), today announced a groundbreaking world record in data rate achieved using sub-THz frequencies.
Priority Software Announces the New, Game-Changing aiERP
06/12/2025 | Priority SoftwarePriority Software Ltd., a leading global provider of ERP and business management software announces its revolutionary aiERP, leveraging the power of AI to transform business operations.