Wearable Sensors Can Tell When You Are Getting Sick
January 13, 2017 | Stanford UniversityEstimated reading time: 6 minutes
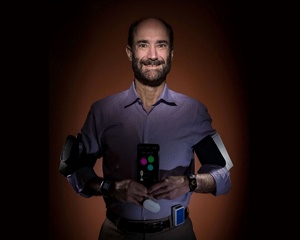
Wearable sensors that monitor heart rate, activity, skin temperature and other variables can reveal a lot about what is going on inside a person, including the onset of infection, inflammation and even insulin resistance, according to a study by researchers at the Stanford University School of Medicine.
Geneticist Michael Snyder was wearing seven biosensors collecting data about his health when he noticed changes in his heart rate and oxygen level during a flight. When he later developed a fever, he suspected he had been infected with Lyme disease. Subsequent tests confirmed his suspicion. Steve Fisch
An important component of the ongoing study is to establish a range of normal, or baseline, values for each person in the study and when they are ill. “We want to study people at an individual level,” said Michael Snyder, PhD, professor and chair of genetics.
Snyder is the senior author of the study, which was published online Jan. 12 in PLOS Biology. Postdoctoral scholars Xiao Li, PhD, and Jessilyn Dunn, PhD, and software engineer Denis Salins share lead authorship.
Altogether, the team collected nearly 2 billion measurements from 60 people, including continuous data from each participant’s wearable biosensor devices and periodic data from laboratory tests of their blood chemistry, gene expression and other measures. Participants wore between one and seven commercially available activity monitors and other monitors that collected more than 250,000 measurements a day. The team collected data on weight; heart rate; oxygen in the blood; skin temperature; activity, including sleep, steps, walking, biking and running; calories expended; acceleration; and even exposure to gamma rays and X-rays.
“I was very impressed with all the data that was collected,” said Eric Topol, MD, professor of genomics at the Scripps Research Institute, who was not involved in the study. “There’s a lot here — a lot of sensors and a lot of different data on each person.”
The study demonstrated that, given a baseline range of values for each person, it is possible to monitor deviations from normal and associate those deviations with environmental conditions, illness or other factors that affect health. Distinctive patterns of deviation from normal seem to correlate with particular health problems. Algorithms designed to pick up on these patterns of change could potentially contribute to clinical diagnostics and research.
The work is an example of Stanford Medicine’s focus on precision health, whose goal is to anticipate and prevent disease in the healthy and to precisely diagnose and treat disease in the ill.
An unexpected diagnosis
On a long flight to Norway for a family vacation last year, Snyder noticed changes in his heart rate and blood oxygen levels. As one of the 60 participants in the digital health study, he was wearing seven biosensors. From previous trips, Snyder knew that his oxygen levels normally dropped during airplane flights and that his heart rate increased at the beginning of a flight — as occurred in other participants. But the values typically returned to normal over the course of a long flight and after landing. This time, his numbers didn’t return to baseline. Something was up, and Snyder wasn’t completely surprised when he went on to develop a fever and other signs of illness.
The fact that you can pick up infections by monitoring before they happen is very provocative.
Two weeks earlier, he’d been helping his brother build a fence in rural Massachusetts, so his biggest concern was that he might have been bitten by a tick and infected with Lyme disease. In Norway, Snyder persuaded a doctor to give him a prescription for doxycycline, an antibiotic known to combat Lyme disease. Subsequent tests confirmed that Snyder had indeed been infected with the Lyme microorganism.
Snyder was impressed that the wearable biosensors picked up the infection before he even knew he was sick. “Wearables helped make the initial diagnosis,” he said. Subsequent data analysis confirmed his suspicion that the deviations from normal heart rate and oxygen levels on the flight to Norway had indeed been quite abnormal.
“The fact that you can pick up infections by monitoring before they happen is very provocative,” said Topol.
Page 1 of 2
Suggested Items
New Database of Materials Accelerates Electronics Innovation
05/02/2025 | ACN NewswireIn a collaboration between Murata Manufacturing Co., Ltd., and the National Institute for Materials Science (NIMS), researchers have built a comprehensive new database of dielectric material properties curated from thousands of scientific papers.
IT Distribution Records Strong Revenue Growth in Q1 Fueled by Personal Computing Purchases Amidst Tariff Uncertainty
05/02/2025 | IDCSales through distribution in North America posted a second consecutive quarter of growth in the first quarter of 2025. Distributor Revenues came in at $19.9B which is a 7.6% increase year-over-year, according to the International Data Corporation (IDC) North America Distribution Track e r (NADT).
INEMI Smart Manufacturing Tech Topic Series: Enhancing Yield and Quality with Explainable AI
05/02/2025 | iNEMIIn semiconductor manufacturing, the ability to analyze vast amounts of high-dimensional data is critical for ensuring product quality and optimizing wafer yield.
Nolan's Notes: The Next Killer App in Component Manufacturing
05/02/2025 | Nolan Johnson -- Column: Nolan's NotesFor quite a while, I’ve been wondering what the next “killer app” will be in electronics manufacturing and why it has been so long since the last disruptive change in EMS. I believe the answer lies in artificial intelligence, which has exploded as the next disruptor.
Keysight EDA, Intel Foundry Collaborate on EMIB-T Silicon Bridge Technology for Next-Generation AI and Data Center Solutions
04/30/2025 | BUSINESS WIREKeysight Technologies, Inc. announced a collaboration with Intel Foundry to support Embedded Multi-die Interconnect Bridge-T (EMIB-T) technology, a cutting-edge innovation aimed at improving high-performance packaging solutions for artificial intelligence (AI) and data center markets in addition to the support of Intel 18A process node.