MagnetPredictor: Predicting the Magnetic Properties of Materials
September 6, 2018 | Fraunhofer-GesellschaftEstimated reading time: 3 minutes
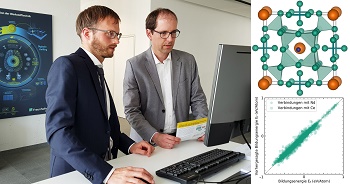
Permanent magnets used in electric cars and wind turbines currently contain rare earth metals. Reducing the amount of these elements in magnets is important, as mining them is harmful both to health and the environment. Researchers have now developed a new machine learning tool to assist in quickly and easily predicting the ferromagnetic crystal properties of novel material compositions.
Image Caption: The MagnetPredictor tool can predict the magnetic properties of combinations of rare earth elements, transition metals and other elements.
Renewable energy is a key technology for the future. However, both electric cars and wind turbines require large and strong permanent magnets. The inherent problem is that high-performance magnetic materials contain 12−17% rare earth elements, chiefly neodymium and samarium, but also dysprosium and terbium. The source of these elements is, almost exclusively, China. In addition, miners who extract these raw materials usually work under health-hazardous conditions, and the process damages the environment. Not surprisingly, materials researchers have set their sights for years on finding alternatives to the rare earth metals in permanent magnets. On the whole, the standard method is “trial and error:” which elemental compositions have worked well in the past, and which might work equally well in the future? Testing like this is a costly and time-consuming undertaking.
Collecting Candidates Using Computer Simulation
Researchers at the Fraunhofer Institute for Mechanics of Materials IWM in Freiburg are pioneering an alternative, more effective approach. “We have developed a high-throughput computer simulation method to systematically and rapidly test a large number of materials as candidates for permanent magnets,” explains Dr. Johannes Möller, a research scientist in the Material Design business unit at Fraunhofer IWM. “Our method isn’t to consider which particular percentage of manganese, cobalt or boron might be viable, but to let the computer simulate many conceivable variants.” This combinatorial approach can filter out promising compositions to create a collection of reasonable theoretical candidates that can then be systematically investigated. This considerably narrows things down compared to conventional trial and error methods. “In principle, this approach is not restricted to magnetic properties, but can also be applied to other material properties,” Möller emphasizes.
The computer only needs a limited amount of information to perform the simulation: just the crystal structure of the magnetic material and the chemical elements it contains. “Everything else depends on the physical context,” Möller clarifies. When it comes to the crystal structure, the researchers are banking on crystal lattices in which just one in every fourteen atoms is a rare earth metal element – corresponding to only seven percent. The team has checked how successful the simulation is using known magnetic materials. By successfully identifying the known properties of such materials, they have demonstrated the simulation can successfully predict the magnetism of novel materials. What is equally important, however, is the magnetic anisotropy constant. This value is a measure of how easy or difficult it is to reverse the polarity of a magnetic material by applying a magnetic field. “Being able to predict this value is a huge challenge for computer-aided magnetic materials science,” says Möller. However, the scientists can instead calculate a semi-quantitative value; in other words, the simulation can systematically predict a value for magnetic anisotropy that is qualitatively rather than quantitatively precise. The simulation, for instance, can show that material X is able to withstand magnetic fields seven times stronger than material Y.
Machine Learning Fills the Gaps
The team can now employ their data on the magnetic properties of materials in a further, and larger, step. “The simulation provides us with several thousand to ten thousand candidates. However, there are millions or even billions of potential elemental compositions and combinations,” Möller explains. “Using machine learning methods, we are able to fill in the large gaps between the simulated and theoretical figures.” The researchers can also reverse the process to optimize materials. To do this, they specify the minimum requirements for a material, for example the magnetic strength or the anisotropy, along with the chemical elements they hope to employ, for instance specifying “use cheap copper rather than rare and expensive cobalt.” An optimization algorithm then provides the best possible elemental composition of the material, using the material model calculated by machine learning from the material data.
The team has developed a user-friendly web tool to make the software easier to use. This allows users to enter the target properties and source materials. The tool then provides information on the magnetic properties and raw material costs. The implemented optimization algorithm will soon be available.
Suggested Items
Elephantech: For a Greener Tomorrow
04/16/2025 | Marcy LaRont, PCB007 MagazineNobuhiko Okamoto is the global sales and marketing manager for Elephantech Inc., a Japanese startup with a vision to make electronics more sustainable. The company is developing a metal inkjet technology that can print directly on the substrate and then give it a copper thickness by plating. In this interview, he discusses this novel technology's environmental advantages, as well as its potential benefits for the PCB manufacturing and semiconductor packaging segments.
Trouble in Your Tank: Organic Addition Agents in Electrolytic Copper Plating
04/15/2025 | Michael Carano -- Column: Trouble in Your TankThere are numerous factors at play in the science of electroplating or, as most often called, electrolytic plating. One critical element is the use of organic addition agents and their role in copper plating. The function and use of these chemical compounds will be explored in more detail.
IDTechEx Highlights Recyclable Materials for PCBs
04/10/2025 | IDTechExConventional printed circuit board (PCB) manufacturing is wasteful, harmful to the environment and energy intensive. This can be mitigated by the implementation of new recyclable materials and technologies, which have the potential to revolutionize electronics manufacturing.
Connect the Dots: Stop Killing Your Yield—The Hidden Cost of Design Oversights
04/03/2025 | Matt Stevenson -- Column: Connect the DotsI’ve been in this industry long enough to recognize red flags in PCB designs. When designers send over PCBs that look great on the computer screen but have hidden flaws, it can lead to manufacturing problems. I have seen this happen too often: manufacturing delays, yield losses, and designers asking, “Why didn’t anyone tell me sooner?” Here’s the thing: Minor design improvements can greatly impact manufacturing yield, and design oversights can lead to expensive bottlenecks. Here’s how to find the hidden flaws in a design and avoid disaster.
Real Time with... IPC APEX EXPO 2025: Tariffs and Supply Chains in U.S. Electronics Manufacturing
04/01/2025 | Real Time with...IPC APEX EXPOChris Mitchell, VP of Global Government Relations for IPC, discusses IPC's concerns about tariffs on copper and their impact on U.S. electronics manufacturing. He emphasizes the complexity of supply chains and the need for policymakers to understand their effects.