Finding Novel Materials for Practical Devices
August 13, 2019 | MITEstimated reading time: 10 minutes
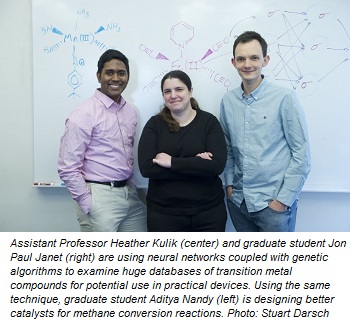
In recent years, machine learning has been proving a valuable tool for identifying new materials with properties optimized for specific applications. Working with large, well-defined data sets, computers learn to perform an analytical task to generate a correct answer and then use the same technique on an unknown data set.
While that approach has guided the development of valuable new materials, they’ve primarily been organic compounds, notes Heather Kulik PhD ’09, an assistant professor of chemical engineering. Kulik focuses instead on inorganic compounds — in particular, those based on transition metals, a family of elements (including iron and copper) that have unique and useful properties. In those compounds — known as transition metal complexes — the metal atom occurs at the center with chemically bound arms, or ligands, made of carbon, hydrogen, nitrogen, or oxygen atoms radiating outward.
Transition metal complexes already play important roles in areas ranging from energy storage to catalysis for manufacturing fine chemicals — for example, for pharmaceuticals. But Kulik thinks that machine learning could further expand their use. Indeed, her group has been working not only to apply machine learning to inorganics — a novel and challenging undertaking — but also to use the technique to explore new territory. “We were interested in understanding how far we could push our models to do discovery — to make predictions on compounds that haven’t been seen before,” says Kulik.
Sensors and computers
For the past four years, Kulik and Jon Paul Janet, a graduate student in chemical engineering, have been focusing on transition metal complexes with “spin” — a quantum mechanical property of electrons. Usually, electrons occur in pairs, one with spin up and the other with spin down, so they cancel each other out and there’s no net spin. But in a transition metal, electrons can be unpaired, and the resulting net spin is the property that makes inorganic complexes of interest, says Kulik. “Tailoring how unpaired the electrons are gives us a unique knob for tailoring properties.”
A given complex has a preferred spin state. But add some energy — say, from light or heat — and it can flip to the other state. In the process, it can exhibit changes in macroscale properties such as size or color. When the energy needed to cause the flip — called the spin-splitting energy — is near zero, the complex is a good candidate for use as a sensor, or perhaps as a fundamental component in a quantum computer.
Chemists know of many metal-ligand combinations with spin-splitting energies near zero, making them potential “spin-crossover” (SCO) complexes for such practical applications. But the full set of possibilities is vast. The spin-splitting energy of a transition metal complex is determined by what ligands are combined with a given metal, and there are almost endless ligands from which to choose. The challenge is to find novel combinations with the desired property to become SCOs — without resorting to millions of trial-and-error tests in a lab.
Translating molecules into numbers
The standard way to analyze the electronic structure of molecules is using a computational modeling method called density functional theory, or DFT. The results of a DFT calculation are fairly accurate — especially for organic systems — but performing a calculation for a single compound can take hours, or even days. In contrast, a machine learning tool called an artificial neural network (ANN) can be trained to perform the same analysis and then do it in just seconds. As a result, ANNs are much more practical for looking for possible SCOs in the huge space of feasible complexes.
Because an ANN requires a numerical input to operate, the researchers’ first challenge was to find a way to represent a given transition metal complex as a series of numbers, each describing a selected property. There are rules for defining representations for organic molecules, where the physical structure of a molecule tells a lot about its properties and behavior. But when the researchers followed those rules for transition metal complexes, it didn’t work. “The metal-organic bond is very tricky to get right,” says Kulik. “There are unique properties of the bonding that are more variable. There are many more ways the electrons can choose to form a bond.” So the researchers needed to make up new rules for defining a representation that would be predictive in inorganic chemistry.
Using machine learning, they explored various ways of representing a transition metal complex for analyzing spin-splitting energy. The results were best when the representation gave the most emphasis to the properties of the metal center and the metal-ligand connection and less emphasis to the properties of ligands farther out. Interestingly, their studies showed that representations that gave more equal emphasis overall worked best when the goal was to predict other properties, such as the ligand-metal bond length or the tendency to accept electrons.
Testing the ANN
As a test of their approach, Kulik and Janet — assisted by Lydia Chan, a summer intern from Troy High School in Fullerton, California — defined a set of transition metal complexes based on four transition metals — chromium, manganese, iron, and cobalt — in two oxidation states with 16 ligands (each molecule can have up to two). By combining those building blocks, they created a “search space” of 5,600 complexes — some of them familiar and well-studied, and some of them totally unknown.
In previous work, the researchers had trained an ANN on thousands of compounds that were well-known in transition metal chemistry. To test the trained ANN’s ability to explore a new chemical space to find compounds with the targeted properties, they tried applying it to the pool of 5,600 complexes, 113 of which it had seen in the previous study.
The result was the plot labeled "Figure 1" in the slideshow above, which sorts the complexes onto a surface as determined by the ANN. The white regions indicate complexes with spin-splitting energies within 5 kilo-calories per mole of zero, meaning that they are potentially good SCO candidates. The red and blue regions represent complexes with spin-splitting energies too large to be useful. The green diamonds that appear in the inset show complexes that have iron centers and similar ligands — in other words, related compounds whose spin-crossover energies should be similar. Their appearance in the same region of the plot is evidence of the good correspondence between the researchers’ representation and key properties of the complex.
But there’s one catch: Not all of the spin-splitting predictions are accurate. If a complex is very different from those on which the network was trained, the ANN analysis may not be reliable — a standard problem when applying machine learning models to discovery in materials science or chemistry, notes Kulik. Using an approach that looked successful in their previous work, the researchers compared the numeric representations for the training and test complexes and ruled out all the test complexes where the difference was too great.
Page 1 of 2
Suggested Items
RF PCB Design Tips and Tricks
05/08/2025 | Cherie Litson, EPTAC MIT CID/CID+There are many great books, videos, and information online about designing PCBs for RF circuits. A few of my favorite RF sources are Hans Rosenberg, Stephen Chavez, and Rick Hartley, but there are many more. These PCB design engineers have a very good perspective on what it takes to take an RF design from schematic concept to PCB layout.
Trouble in Your Tank: Causes of Plating Voids, Pre-electroless Copper
05/09/2025 | Michael Carano -- Column: Trouble in Your TankIn the business of printed circuit fabrication, yield-reducing and costly defects can easily catch even the most seasoned engineers and production personnel off guard. In this month’s column, I’ll investigate copper plating voids with their genesis in the pre-plating process steps.
Elephantech: For a Greener Tomorrow
04/16/2025 | Marcy LaRont, PCB007 MagazineNobuhiko Okamoto is the global sales and marketing manager for Elephantech Inc., a Japanese startup with a vision to make electronics more sustainable. The company is developing a metal inkjet technology that can print directly on the substrate and then give it a copper thickness by plating. In this interview, he discusses this novel technology's environmental advantages, as well as its potential benefits for the PCB manufacturing and semiconductor packaging segments.
Trouble in Your Tank: Organic Addition Agents in Electrolytic Copper Plating
04/15/2025 | Michael Carano -- Column: Trouble in Your TankThere are numerous factors at play in the science of electroplating or, as most often called, electrolytic plating. One critical element is the use of organic addition agents and their role in copper plating. The function and use of these chemical compounds will be explored in more detail.
IDTechEx Highlights Recyclable Materials for PCBs
04/10/2025 | IDTechExConventional printed circuit board (PCB) manufacturing is wasteful, harmful to the environment and energy intensive. This can be mitigated by the implementation of new recyclable materials and technologies, which have the potential to revolutionize electronics manufacturing.