Machine Learning Predicts Mechanical Properties of Porous Materials
May 16, 2019 | University of CambridgeEstimated reading time: 2 minutes
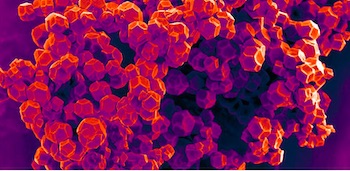
Machine learning can be used to predict the properties of a group of materials which, according to some, could be as important to the 21st century as plastics were to the 20th.
Researchers have used machine learning techniques to accurately predict the mechanical properties of metal-organic frameworks (MOFs), which could be used to extract water from the air in the desert, store dangerous gases or power hydrogen-based cars.
The researchers, led by the University of Cambridge, used their machine learning algorithm to predict the properties of more than 3000 existing MOFs, as well as MOFs which are yet to be synthesised in the laboratory.
The results, published in the inaugural edition of the Cell Press journal Matter, could be used to significantly speed up the way materials are characterised and designed at the molecular scale.
MOFs are self-assembling 3D compounds made of metallic and organic atoms connected together. Like plastics, they are highly versatile, and can be customised into millions of different combinations. Unlike plastics, which are based on long chains of polymers that grow in only one direction, MOFs have orderly crystalline structures that grow in all directions.
This crystalline structure means that MOFs can be made like building blocks: individual atoms or molecules can be switched in or out of the structure, a level of precision that is impossible to achieve with plastics.
The structures are highly porous with massive surface area: a MOF the size of a sugar cube laid flat would cover an area the size of six football fields. Perhaps somewhat counterintuitively however, MOFs make highly effective storage devices. The pores in any given MOF can be customised to form a perfectly-shaped storage pocket for different molecules, just by changing the building blocks.
“That MOFs are so porous makes them highly adaptable for all kinds of different applications, but at the same time their porous nature makes them highly fragile,” said Dr David Fairen-Jimenez from Cambridge’s Department of Chemical Engineering and Biotechnology, who led the research.
MOFs are synthesised in powder form, but in order to be of any practical use, the powder is put under pressure and formed into larger, shaped pellets. Due to their porosity, many MOFs are crushed in this process, wasting both time and money.
To address this problem, Fairen-Jimenez and his collaborators from Belgium and the US developed a machine learning algorithm to predict the mechanical properties of thousands of MOFs, so that only those with the necessary mechanical stability are manufactured.
The researchers used a multi-level computational approach in order to build an interactive map of the structural and mechanical landscape of MOFs. First, they used high-throughput molecular simulations for 3,385 MOFs. Secondly, they developed a freely-available machine learning algorithm to automatically predict the mechanical properties of existing and yet-to-be-synthesised MOFs.
“We are now able to explain the landscape for all the materials at the same time,” said Fairen-Jimenez. “This way, we can predict what the best material would be for a given task.”
The researchers have launched an interactive website where scientists can design and predict the performance of their own MOFs. Fairen-Jimenez says that the tool will help to close the gap between experimentalists and computationalists working in this area. “It allows researchers to access the tools they need in order to work with these materials: it simplifies the questions they need to ask,” he said.
Suggested Items
IPC Releases Version 2.0 of IPC-2591, Connected Factory Exchange, with Expanded Device Coverage and Smarter Data
04/23/2025 | IPCIPC announces the release of IPC-2591, Connected Factory Exchange (CFX), Version 2.0, the global standard for plug-and-play, machine-to-machine, and machine-to-system communication for digital manufacturing.
UHDI Fundamentals: UHDI Drives Unique IoT Innovation in Farming
04/22/2025 | Anaya Vardya, American Standard CircuitsThe combination of UHDI's high-bandwidth capabilities and IoT's real-time data processing can lead to more efficient, immersive, and smarter IoT systems. This convergence of two revolutionary technologies is enabling quantum advancements in some very “unconventional” applications. The typical discussions around UHDI focus on our standard electronics industry market segments like milaero, medical, consumer electronics, etc. IoT is all about machines talking to other machines, machine learning, and artificial intelligence, but again, typically applied in our PCB and assembly operations.
SAA and ASMPT Honored with Technica USA’s 2024 Supplier Alliance Awards
04/15/2025 | Technica USATechnica USA was pleased to announce the recipients of its 2024 Supplier Alliance Awards, presented at the 2025 IPC/APEX Expo in Anaheim, California. These prestigious awards recognize outstanding suppliers in the PCB fabrication and PCB assembly sectors, honoring those who go above and beyond in aligning their resources, initiatives, and expertise with Technica’s mission to achieve unparalleled success.
ASMPT Demonstrates Technology Leadership in SMT Assembly
04/09/2025 | ASMPTThe rapid development of artificial intelligence is driving the demand for high-performance processors and placing great demands on electronics manufacturing.
Aegis Software and Hanwha Partner to Deliver SaaS-Based SMT Programming
04/09/2025 | Aegis SoftwareAegis Software, a global provider of Manufacturing Operations Management Software (MOM/MES) software, today announced a partnership with Hanwha Semitech Americas, a leader in Surface Mount Technology (SMT) and electronics assembly solutions. Through this partnership, Hanwha SMT customers in the U.S. will have the option to leverage Aegis’ FactoryLogix® Machine Programmer solution, available as a cloud-based SaaS offering—enabling automated machine programming, faster new product introduction (NPI), and improved production efficiency without the need for on-premises infrastructure.