Using Machine Learning to Create More Capable Capacitors
July 3, 2019 | UC San DiegoEstimated reading time: 3 minutes
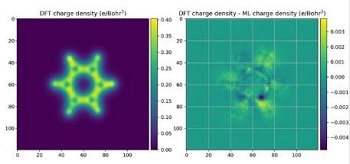
Capacitors, given their high energy output and recharging speed, could play a major role in powering the machines of the future, from electric cars to cell phones. However, the biggest hurdle for capacitors as energy storage devices is that they store much less energy than a similar-sized battery.
Image Caption: Scientists at Georgia Tech are using machine learning with supercomputers to analyze the electronic structure of materials to ultimately find ways to build more capable capacitors. (Left) Density functional theory (DFT) charge density of a molecular dynamics snapshot of a benzene. (Right) Charge density difference between machine learning prediction and DFT for the same benzene structure. Image: Rampi Ramprasad, Georgia Tech
Researchers at Georgia Institute of Technology (Georgia Tech) are tackling that problem in a novel way by using supercomputers and machine learning techniques to ultimately find ways to build more capable capacitors.
The method was described in Nature Partner Journal’s Computational Materials, published in February 2019. The study involved teaching a computer to analyze at the atomic level two materials, aluminum and polyethylene, which are used to make some capacitors.
The researchers focused on finding a way to more quickly analyze the electronic structure of the capacitor materials, looking for features that could affect performance. “The electronics industry wants to know the electronic properties and structure of all of the materials they use to produce devices, including capacitors,” said Rampi Ramprasad, a professor in Georgia Tech’s School of Materials Science and Engineering.
For example, polyethylene is a very good insulator with a large band gap, the energy range forbidden to electrical charge carriers. But if it has a defect, unwanted charge carriers are allowed into the band gap, reducing efficiency, he said.
“In order to understand where the defects are and what role they play, we need to compute the entire atomic structure, something that so far has been extremely difficult,” said Ramprasad. “The current method of analyzing those materials using quantum mechanics is so slow that it limits how much analysis can be performed at any given time.”
Ramprasad and his colleagues used machine learning to help develop new materials. Here they used a sample of data created from a quantum mechanical analysis of aluminum and polyethylene as an input to teach a powerful computer how to simulate that analysis.
Analyzing the electronic structure of a material with quantum mechanics involves solving the Kohn-Sham equation of density functional theory, which generates data on wave functions and energy levels. That data is then used to compute the total potential energy of the system and atomic forces.
The researchers used the Comet supercomputer at the San Diego Supercomputer Center, an Organized Research Unit of the University of California San Diego for early calculations; and the Stampede2 supercomputer at the Texas Advanced Computing Center, at the University of Texas at Austin, for the later stages of this research. Both systems are funded by the National Science Foundation under multi-year awards.
“In the work leading up to the study, we used Comet extensively for high-throughput polymer electronic property calculation, such as the effect of polymer morphology on the bandage of polymers,” said study co-author Deepak Kamal, a graduate student advised by Ramprasad at the Georgia Tech School of Materials Science and Engineering. “We used Comet because it was fast and efficient at handling large number and quantities of calculations.”
Using the new machine learning method developed by Ramprasad and colleagues produced similar results several orders of magnitude faster than using the conventional technique based on quantum mechanics.
“This unprecedented speed-up in computational capability will allow us to design electronic materials that are superior to what is currently out there,” Ramprasad said. “Basically, we can say, ‘here are defects with this material that will really diminish the efficiency of its electronic structure.’ Once we can address such aspects efficiently, we can better design electronic devices.”
While the study focused on aluminum and polyethylene, machine learning could be used to analyze the electronic structure of a wider range of materials. Beyond analyzing electronic structure, other aspects of material structure now analyzed by quantum mechanics could also be hastened by the machine learning approach, Ramprasad said.
“In part we selected aluminum and polyethylene because they are components of a capacitor,” he explained. “But we also demonstrated that one can use this method for vastly different materials, such as metals that are conductors and polymers that are insulators.”
The faster processing allowed by the machine learning method would also enable researchers to more quickly simulate how modifications to a material will impact its electronic structure, potentially revealing new ways to improve its efficiency.
Added Kamal: “Supercomputing systems allow high-throughputcomputing which enables us to create vastdatabases of knowledge about various materialsystems. This knowledge can then be utilized tofind the best material for a specific application.”
Suggested Items
Taiwan's PCB Industry Chain Is Expected to Grow Steadily by 5.8% Annually in 2025
05/05/2025 | TPCAAccording to an analysis report jointly released by the Taiwan Printed Circuit Association (TPCA) and the Industrial Technology Research Institute's International Industrial Science Institute, the total output value of Taiwan's printed circuit (PCB) industry chain will reach NT$1.22 trillion in 2024, with an annual growth rate of 8.1%.
New Database of Materials Accelerates Electronics Innovation
05/05/2025 | ACN NewswireIn a collaboration between Murata Manufacturing Co., Ltd., and the National Institute for Materials Science (NIMS), researchers have built a comprehensive new database of dielectric material properties curated from thousands of scientific papers.
DuPont Exceeds Quarterly Profit Expectations as Electronics Segment Benefits from Semiconductor Demand
05/05/2025 | I-Connect007 Editorial TeamDuPont reported higher-than-expected earnings for the first quarter of 2025, supported by increased demand in its electronics and industrial segments. The company’s adjusted earnings per share came in at 79 cents, surpassing the average analyst estimate of 65 cents per share, according to data from LSEG.
SEMICON Europa 2025 Call for Abstracts Opens for Advanced Packaging Conference and MEMS & Imaging Summit
05/05/2025 | SEMISEMI Europe announced the opening of the call for abstracts for SEMICON Europa 2025, to be held November 18-21 at Messe München in Munich, Germany. Selected speakers will share their expertise at the Advanced Packaging Conference (APC), MEMS & Imaging Sensors Summit, and during presentations on the show floor.
New Database of Materials Accelerates Electronics Innovation
05/02/2025 | ACN NewswireIn a collaboration between Murata Manufacturing Co., Ltd., and the National Institute for Materials Science (NIMS), researchers have built a comprehensive new database of dielectric material properties curated from thousands of scientific papers.