First Programmable Memristor Computer Aims to Bring AI Processing Down from the Cloud
July 18, 2019 | Michigan State UniversityEstimated reading time: 4 minutes
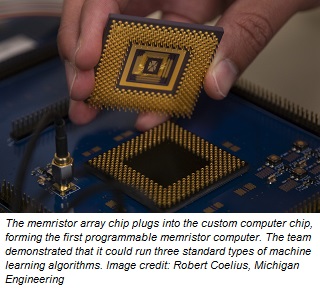
The memristor array chip plugs into the custom computer chip, forming the first programmable memristor computer. The team demonstrated that it could run three standard types of machine learning algorithms. Image credit: Robert Coelius, Michigan Engineering.
ANN ARBOR—The first programmable memristor computer—not just a memristor array operated through an external computer—has been developed at the University of Michigan.
It could lead to the processing of artificial intelligence directly on small, energy-constrained devices such as smartphones and sensors. A smartphone AI processor would mean that voice commands would no longer have to be sent to the cloud for interpretation, speeding up response time.
“Everyone wants to put an AI processor on smartphones, but you don’t want your cell phone battery to drain very quickly,” said Wei Lu, U-M professor of electrical and computer engineering and senior author of the study in Nature Electronics.
In medical devices, the ability to run AI algorithms without the cloud would enable better security and privacy.
Why Memristors are Good for Machine Learning
The key to making this possible could be an advanced computer component called the memristor. This circuit element, an electrical resistor with a memory, has a variable resistance that can serve as a form of information storage. Because memristors store and process information in the same location, they can get around the biggest bottleneck for computing speed and power: the connection between memory and processor.
This is especially important for machine-learning algorithms that deal with lots of data to do things like identify objects in photos and videos—or predict which hospital patients are at higher risk of infection. Already, programmers prefer to run these algorithms on graphical processing units rather than a computer’s main processor, the central processing unit.
“GPUs and very customized and optimized digital circuits are considered to be about 10-100 times better than CPUs in terms of power and throughput.” Lu said. “Memristor AI processors could be another 10-100 times better.”
GPUs perform better at machine learning tasks because they have thousands of small cores for running calculations all at once, as opposed to the string of calculations waiting their turn on one of the few powerful cores in a CPU.
A memristor array takes this even further. Each memristor is able to do its own calculation, allowing thousands of operations within a core to be performed at once. In this experimental-scale computer, there were more than 5,800 memristors. A commercial design could include millions of them.
Memristor arrays are especially suited to machine learning problems. The reason for this is the way that machine learning algorithms turn data into vectors—essentially, lists of data points. In predicting a patient’s risk of infection in a hospital, for instance, this vector might list numerical representations of a patient’s risk factors.
Then, machine learning algorithms compare these “input” vectors with “feature” vectors stored in memory. These feature vectors represent certain traits of the data (such as the presence of an underlying disease). If matched, the system knows that the input data has that trait. The vectors are stored in matrices, which are like the spreadsheets of mathematics, and these matrices can be mapped directly onto the memristor arrays.
What’s more, as data is fed through the array, the bulk of the mathematical processing occurs through the natural resistances in the memristors, eliminating the need to move feature vectors in and out of the memory to perform the computations. This makes the arrays highly efficient at complicated matrix calculations. Earlier studies demonstrated the potential of memristor arrays for speeding up machine learning, but they needed external computing elements to function.
Wei Lu stands with first author Seung Hwan Lee, an electrical engineering PhD student, who holds the memristor array. Image credit: Robert Coelius, Michigan Engineering
Page 1 of 2
Suggested Items
2025 COMPUTEX Compal Unveils 'The Race is On' Strategy During Forum Session
05/23/2025 | Compal Electronics Inc.As AI models and computing density continue to grow at an exponential rate, the data center industry is entering a new era where cooling capacity has become the next competitive threshold.
HBM4 Raises the Bar on Manufacturing Complexity, Premium Expected to Exceed 30%
05/22/2025 | TrendForceTrendForce's latest findings reveal that demand for AI servers continues to accelerate the development of HBM technologies, with the three major suppliers actively advancing their HBM4 product roadmaps.
SEMI, Purdue University Launch AI and Data Analysis Online Courses
05/22/2025 | SEMISEMI, the industry association serving the global semiconductor and electronics design and manufacturing supply chain, today announced it has partnered with Purdue University to launch an online course series focused on artificial intelligence (AI) and data analysis techniques for the semiconductor industry.
ASMPT Presents Central Platform for Data Exchange in Electronics Manufacturing
05/21/2025 | ASMPTWith WORKS Integration, ASMPT SMT Solutions, the market and technology leader in SMT, provides a central integration platform through which all its hardware and software solutions can communicate with each other.
Infineon to Revolutionize Power Delivery Architecture for Future AI Server Racks with NVIDIA
05/21/2025 | InfineonInfineon Technologies AG is revolutionizing the power delivery architecture required for future AI data centers. In collaboration with NVIDIA, Infineon is developing the next generation of power systems based on a new architecture with central power generation of 800 V high-voltage direct current (HVDC).