Estimated reading time: 6 minutes
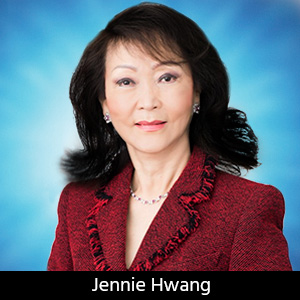
SMT Prospects and Perspectives: AI Opportunities, Challenges, and Possibilities, Part 1
In this installment of my artificial intelligence (AI) series, I will touch on the key foundational technologies that propel and drive the development and deployment of AI, with special consideration of electronics packaging and assembly.
The objectives of the series:
- Build and stay in the knowledge zone
- Spur innovative ideas and inspire new vistas for new opportunities
- Highlight what it takes to achieve AI with justified confidence and trust
- Achieve a balance between AI’s omnipotent power and its potential downsides
Leverage AI as a virtual tool to facilitate an individual’s job efficiency and effectiveness and future job prospects, as well as the enterprise business growth
Breakthroughs and Transformational Technologies
Since the discovery of the electron in 1897 by Joseph John Thomson, striking breakthroughs of the 20th and 21st centuries include:
- Invention of the transistor in 1947
- Introduction of the microprocessor in 1972
- Official birth of the internet in 1983
- Internet-enabled hardware and applications during the decade of 1990s
- AI development in the decade of 2010s
Introduction of AI ChatGPT-4 by OpenAI in 2023
Based on these breakthrough technologies, many products and services have been developed that improve the quality of human life and spur global prosperity—and it all came from the discovery of that tiny unit called an electron.
Operating AI demands the use of heavy-load hardware that processes algorithms, runs the models, and keeps data flowing. These bandwidth-hungry applications necessitate higher-speed data transfer, which opens a crucial role for photons by taking advantage of the speed of light to deliver greater bandwidth and lower latency and power. Hardware components typically will connect via copper interconnects, while the connections between the racks in data centers often use optical fiber. CPUs and GPUs also use optical interconnects for optical signals.
Both electrons and photons will play an increased role. AI will drive the need for near-packaged optics with high-performance PCB substrates (or an interposer) on the host board. Co-packaged optics, a single-package integration of electronic and photonic dies, or photonic integrated circuits (PICs) are expected to play a pivotal role.
AI Market and Hardware
To AI, high performance hardware is indispensable, particularly with computing chips. As AI becomes embedded in all sectors of industry and all aspects of daily life and business, the biggest winners so far are hardware manufacturers: 80% of AI servers use GPUs and it’s expected to grow to 90%. In addition to GPU, the required pairing memory puts high demand for high bandwidth memory (HBM). The advent of generative AI further thrusts accelerated computing, which uses GPUs along with CPUs to meet augmented performances.
Although the estimated forecast of the future AI market varies, according to PwC1, AI could contribute more than $15 trillion to the global economy by 2030. Most agree that the impact of AI adoption could be greater than the inventions of the internet, mobile broadband, and the smartphone combined.
AI Historical Milestones
AI is not a new term. John McCarthy coined “artificial intelligence” and held the first AI conference in 1956. “Shakey the Robot,” the first general-purpose mobile robot, was built in 1969.
In the succeeding decades, AI went through a roller coaster ride of successes and setbacks until the 2010s, when key events, including the introduction of big data and machine learning (ML), created an age in which machines have the capacity to collect and process huge sums of information too cumbersome for a person to process. Other pace-setting technologies—deep learning and neural network—were introduced in 2010, with GAN in 2014, and transformer in 2017.
The 2020s have been when AI “finally” gained traction, especially with the introduction of generative AI, the release of ChatGPT on Nov. 30, 2022, and the phenomenal ChatGPT-4 on March 14, 2023. It feels like AI has suddenly become a global phenomenon. The rest is history.
AI Bedrock Technologies
Generally speaking, AI is a digital technology that mimics the intellectual, analytical, and creative ability of humans, largely by absorbing and finding patterns in an enormous amount of information and data. AI covers a multitude of technologies, including machine learning (ML), deep learning (DL), neural network (NN), natural language processing (NLP), and their closely-aligned technologies. In one way, AI hierarchy can be shown in Figure 1, exhibiting the interrelations and evolution of these underpinning technologies.
Now I’d like to briefly highlight each technology:
Machine Learning
Machine learning is a technique that collects and analyzes data, looks for patterns, and adjusts its actions accordingly to develop statistical mathematical models. The resulting algorithms allow software applications to predict outcomes without explicit programming and incorporate intelligence into a machine by automatically learning from the data. A learning algorithm then trains a model to generate a prediction for the response to new data or the test datasets.
There are three types of ML: supervised, unsupervised, and reinforcement.
- Supervised ML is task-driven and requires a data analyst to provide input and a desired output, then determine which variables the model should analyze
- Unsupervised ML is data-driven and does not have labeled data. Its focus is learning more about the data by inferring patterns in the dataset without reference to the known outputs.
- Reinforcement learning uses algorithms that learn from outcomes and decide which action to take next. In reinforcement learning, there is no data input, or desired output but the reinforcement agent decides what to do to perform the given task by learning from its experience with a trial-and-error method to achieve the maximum reward in an environment. An agent learns to make decisions by interacting with an environment and receives feedback in the form of rewards or penalties based on the actions it takes.
In addition to these basic ML techniques, more advanced ML approaches continue to emerge.
ML understands patterns and can instantly see anomalies that fall outside those patterns, making it a valuable tool in myriad applications, ranging from fraud detection and cyber threat detection to manufacturing and supply chain operation.
Deep Learning
Deep learning is a subset of machine learning based on multi-layered neural networks that learn from vast amounts of data. It comprises a series of algorithms trained and run on deep neural networks that mimic the human brain to incorporate intelligence into a machine. Most deep learning methods use neural network architectures, so they are often referred to as deep neural networks. Software architecture (type, number, and organization of the layers) is built empirically following an intuition-based optimization process, with training data in the loop to tune DL model parameters. Training for DL software occurs “atomically” and with strong coupling across all layers of the DL software.
The increased accuracy of DL software requires more complex implementations in which the number of layers, their size (number of neurons), and the amount of data used for training increase enormously.
Generative AI
I tried ChatGPT to see how the bot explains generative AI:
“Generative AI refers to a category of artificial intelligence (AI) that focuses on creating new and original content. It uses models and algorithms to generate data, such as text, images, audios, or even videos, that resemble human-created content. Generative AI models are trained on large datasets and can generate creative and coherent outputs based on the patterns and information that have been learned. They have applications in various fields, including art, language, music, and more.”
A generative AI model, in a mathematical representation implemented as an algorithm, can create something that didn't previously exist by processing a large amount of visual or textual data and then determining what things are most likely to appear near other things using deep learning or neural networks. Programming work goes into creating algorithms that can recognize texts or prompts. It creates output by assessing an enormous corpus of data, responding to prompts with something that falls within the realm of probability as determined by that corpus of data.
Generative AI tools offer the ability to create essays, images, and music in response to simple prompts.
My next column will highlight the foundational technologies behind AI, including the large language model (LLM) and foundation model.
References
1. PwC’s Global Artificial Intelligence Study: Exploiting the AI Revolution, pwc.com.
This column originally appeared in the April 2024 issue of SMT007 Magazine.
More Columns from SMT Perspectives and Prospects
SMT Perspectives and Prospects: The AI Era, Part 3: LLMs, SLMs, and Foundation ModelsSMT Perspectives and Prospects: A Dose of Wisdom
SMT Perspectives and Prospects: Critical Materials—A Compelling Case, Part 3
SMT Prospects and Perspectives: AI—A Prelude to Opportunities, Challenges and Possibilities
SMT Perspectives and Prospects: Pearls of Wisdom
SMT Perspectives and Prospects: The Role of Bismuth (Bi) in Electronics, Part 7: A Case Study in Fillet-Lifting
SMT Perspectives & Prospects: Creating a Better World Through Engineering
SMT Perspectives and Prospects: Cybersecurity Requires an Active Approach