-
- News
- Books
Featured Books
- pcb007 Magazine
Latest Issues
Current IssueSales: From Pitch to PO
From the first cold call to finally receiving that first purchase order, the July PCB007 Magazine breaks down some critical parts of the sales stack. To up your sales game, read on!
The Hole Truth: Via Integrity in an HDI World
From the drilled hole to registration across multiple sequential lamination cycles, to the quality of your copper plating, via reliability in an HDI world is becoming an ever-greater challenge. This month we look at “The Hole Truth,” from creating the “perfect” via to how you can assure via quality and reliability, the first time, every time.
In Pursuit of Perfection: Defect Reduction
For bare PCB board fabrication, defect reduction is a critical aspect of a company's bottom line profitability. In this issue, we examine how imaging, etching, and plating processes can provide information and insight into reducing defects and increasing yields.
- Articles
- Columns
- Links
- Media kit
||| MENU - pcb007 Magazine
Estimated reading time: 14 minutes
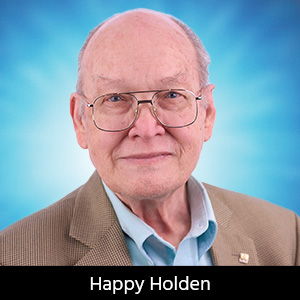
Contact Columnist Form
Learning Theory/Learning Curves
Learning is not instantaneous! Nor is progress made in a steady manner, but at a rate that is typified by one of two basic patterns. The first of the learning curves in Figure 1 shows that, for simple tasks, learning occurs first at a rapid rate and then levels off as the task is mastered. These curves apply not only to individuals, but to manufacturing processes and have especially been used to study and understand the costs over the lifetime of a new product or process.
For complex tasks or material, however, the learning curve is significantly different. Initial progress is slow as the learner develops an understanding of the task, and then accelerates as the material is absorbed. Again, levelling off occurs as the subject is mastered.
Figure 1: Learning curves for different types of material.
In some cases, plateaus will be seen in learning curves. These are caused by factors such as fatigue, poor motivation, loss of interest, or needing time to absorb all the material before progressing to new.
One comment about the learning curve was written by Nick Pearne of PBA Consulting: “Learning curve theory is an anathema to most manufacturing people as a systematized approach to leaving money on the table. The learning curve pricing practices adopted in the 1950s and 1960s by major players in the budding U.S. semiconductor market were more responsible than any other single factor for the financial erosion of the world semiconductor and consumer electronics industries. Billions of dollars in potential profits were sacrificed by pricing from learning curve forecasts to obtain market share, instilling along the way an expectation of dramatic reductions in cost per function.”[1]
Yet there are many applications in which the learning curve approach has real use because, like it or not, in a competitive market environment and, for a wide range of processes, there is always at least a tendency for costs to follow its laws. The learning curve applies in some degree to any process or class of processes in which costs get lower with improving technology. Take as an example, tooling methods: working with a customer builds understanding and standardization; productivity increases as equipment and processes are evolved for that customer; design flaws are detected and corrected; engineering changes decrease over time; and yields improve, reducing rework/rejects.
The degree of fit tends to be excellent for long running processes with low material content and high added value, such as making bicycles, turbine blades or spark plugs. It is probably the worst for processes with extreme and uncontrolled variability. Printed circuit and particularly multilayer processes are certainly somewhere in this arena; the steady erosion of prices which has occurred over time suggests that both experience and market growth are in fact having an effect on cost and therefore on pricing. If such a trend is now in effect, there is no reason to expect that it will not continue into the future.
Learning Theory
This column will not go into details of how learning is achieved, but will summarize some of these theories.
Transformative Learning[2]
Transformative learning holds that the way learners interpret and reinterpret their sense experience is central to making meaning and hence, learning. The theory has two basic kinds of learning: instrumental and communicative learning. Instrumental learning focuses on learning through task-oriented problem solving and determination of cause and effect relationships. Communicative learning involves how individuals communicate their feelings, needs and desires.
Behaviorism [3]
Behaviorism focuses only on the objectively observable aspects of learning and discounts the internal processing that might be associated with the activity. Learning is the acquisition of new behavior through conditioning.
There are two types of possible conditioning:
- Classical conditioning, where the behavior becomes a reflex response to stimulus as in the case of Pavlov's Dogs.
- Operant conditioning, where there is reinforcement of the behavior by a reward or a punishment.
The theory of operant conditioning was developed by B.F. Skinner and is known as Radical Behaviorism. The word ‘operant’ refers to the way in which behavior ‘operates on the environment.’ Briefly, a behavior may result either in reinforcement, which increases the likelihood of the behavior recurring, or punishment, which decreases the likelihood of the behavior recurring. It is important to note that, a punisher is not considered to be punishment if it does not result in the reduction of the behavior, and so the terms punishment and reinforcement are determined as a result of the actions. Within this framework, behaviorists are particularly interested in measurable changes in behavior. Educational approaches such as applied behavior analysis, curriculum based measurement, and direct instruction have emerged from this model.
Cognitivism
In cognitive learning, much of the empirical framework of behaviorism was retained even though a new paradigm was begun. Cognitive theories look beyond behavior to explain brain-based learning. Cognitivists consider how human memory works to promote learning. So for example how the natural physiological processes of encoding information into short term memory and long term memory become important to educators.
Once memory theories like the Atknitiveinson-Shiffrin memory model and Baddeley's Working memory model were established as a theoretical framework in Cognitive Psychology, new cognitive frameworks of learning began to emerge during the 1970s, 80s, and 90s. Today researchers are concentrating on topics like Cognitive Load and Information Processing Theory. These theories of learning are very useful as they guide the Instructional design.
Constructivism
Constructivism views learning as a process in which the learner actively constructs or builds new ideas or concepts based upon current and past knowledge. In other words, "learning involves constructing one's own knowledge from one's own experiences." Constructivist learning, therefore, is a very personal endeavor, whereby internalized concepts, rules, and general principles may consequently be applied in a practical real-world context. The teacher acts as a facilitator who encourages students to discover principles for themselves and to construct knowledge by working to solve realistic problems. This is also known as knowledge construction as a social process. We can work to clarify and organize their ideas so we can voice them to others. It gives us opportunities to elaborate on what they learned. We are exposed to the views of others. It enables us to discover flaws and inconsistencies by learning we can get good results. Constructivism itself has many variations, such as active learning, discovery learning, and knowledge building.
Generative Learning Theory[4]
Generative Learning Theory suggests that the learning process is based on the memory that is already stored in our brains, wherein new data is added to our long term memory and becomes part of our knowledge base. The theory of Generative Learning is based on the assumption that the human brain does not just passively observe its environment or the events it experiences, but that it constructs its own perceptions about problems, scenarios, and experiences.
The 4 Key Concepts of Generative Learning Theory
The Generative Learning Theory involves four key concepts that instructional designers can involve (all four of them or just one) depending on the needs of the learner and the learning materials involved.
- Recall occurs when the learner accesses information stored in his long term memory. The primary goal is to encourage learners to learn a content that is based upon facts by using information they have already acquired. Examples of recall techniques might be having the learner repeat information or reviewing it until the concept is fully grasped.
- Integration involves the learner integrating new information with knowledge already collected and stored. The aim is to alter this information into a form, which the learner can more easily remember and access later on. Examples of an integration activity might be having the learner paraphrase the content or creating analogies to explain a concept.
- Organization involves learners linking knowledge they've already collected to new concepts in an effective way. Examples of organization strategies may include creating lists or analyzing the main points of a specific concept.
- Elaboration involves the encouragement of the learner to connect and add new concepts to information that they've already collected, by analyzing the ideas. Examples of elaboration techniques include creative writing, expanding upon a sentence or thought, and visual representations of mental images.
Informal and Post-modern Theories
Informal theories of education deal with more practical breakdown of the learning process. One of these deals with whether learning should take place as a building of concepts toward an overall idea, or the understanding of the overall idea with the details filled in later. Modern thinkers favor the latter, though without any basis in real world research.
Other concerns are the origins of the drive for learning. To this end, many have split off from the mainstream, holding that learning is a primarily self-taught thing and that the ideal learning situation is one that is self-taught. However, real world results indicate that isolated students fail. Social support seems crucial for sustained learning.
Informal learning theory also concerns itself with book vs real-world experience learning. Many consider most schools severely lacking in the second. Newly emerging hybrid instructional models combining traditional classroom and computer enhanced instruction promise the best of both worlds.
Math of the Learning Curves[5]
The Cost vs Quantity Relationship
The theory of the learning curve is based on the simple idea that the time required to perform a task decreases as a worker gains experience. The basic concept is that the time, or cost, of performing a task (e.g., producing a unit of output) decreases at a constant rate as cumulative output doubles. Learning curves are useful for preparing cost estimates, bidding on special orders, setting labor standards, scheduling labor requirements, and setting incentive wage rates.
There are two different learning curve models. The original model was developed by T. P. Wright in 1936 and is referred to as the Cumulative Average Model or Wright's Model. A second model was developed later by a team of researchers at Stanford. Their approach is referred to as the Incremental Unit Time (or Cost) Model or Crawford's Model.
In Wright's Model, the learning curve function is defined as follows: Y = aXb
Where: Y = the cumulative average time (or cost) per unit
X = the cumulative number of units produced
a = time (or cost) required to produce the first unit
b = slope of the function when plotted on log-log paper
= log of the learning rate/log of 2
For an 80% learning curve, b = log 0.8 / log 2 = 0.091 / 0.301 = - 0.32196
The equation used in Crawford's model is as follows: Y = aKb
Where: Y = the incremental unit time (or cost) of the lot midpoint unit
K = the algebraic midpoint of a specific production batch or lot
X (the cumulative number of units produced) can be used in the equation instead of K to find the unit cost of any particular unit, but determining the unit cost of the last unit produced is not useful in determining the cost of a batch of units. The unit cost of each unit in the batch would have to be determined separately. This is obviously not a practical way to solve for the cost of a batch that may involve hundreds, or even thousands of units. A practical approach involves calculating the midpoint of the lot. The unit cost of the midpoint unit is the average unit cost for the lot. Thus, the cost of the lot is found by calculating the cost of the midpoint unit and then multiplying by the number of units in the lot.
Since the relationships are non-linear, the algebraic midpoint requires solving the following equation:
K = [L(1+b)/(N21+b - N11+b)]-1/b
Where: K = the algebraic midpoint of the lot
L = the number of units in the lot
b = log of learning rate/log of 2
N1 = the first unit in the lot minus 1/2
N2 = the last unit in the lot plus 1/2
The following examples are from Nick Pearne’s paper[1]: As mentioned above, the learning curve depends on the fact that experience gained from increased production of any commodity causes a decline in manufacturing costs, and therefore inevitably in prices in a competitive market environment. More exactly, the theory states that every time the quantity of “units” (or “lots”) produced is doubled the corresponding unit (or lot) costs decline by an experience factor F, also known as the learning or improvement ratio. This is determined by the relationship between resources (typically process cost) required to produce double the reference quantity, Qo:
F =C2/C1 (1)
Where C1 is the initial average unit cost and C2 is the average unit cost for double the reference quantity.
From equation (1) it is evident that the higher the value of F, the less change in cost is to be expected due either to process maturity (automation, optimized setup, tooling, yields), or highly customized content, as might be expected from small lot quantities of complex rigid flex assemblies.
For an initial quantity Qo and a final quantity Q the number of “doublings” or fractions thereof for the total quantity produced is given by log(Q/Qo)/log(2). Therefore, the unit cost behavior as a function of quantity can be written as:
C = C1*(F/100) ^ (log(Q/Qo) / log(2)) (2a)
Where C is the unit cost after quantity Q units or lots, C1 is the first unit cost, and F is the experience factor in percent.
A value of 75 for F would be typical of very steep (fast) learning curves, in which process consolidation proceeds rapidly with corresponding reductions in changeover time, improvements in yields, etc. Equation (2a) is awkward to handle since the principal variable, Q, appears in the exponent. It can be rearranged (and simplified) by noting that in general a ^ log(b) is equivalent to b ^ log(a) since either expression can be written as e ^ [log(a)*log(b)]. An alternate and better form for equation (2a) is therefore:
C = C1*q ^ k (2b)
Where q = Q/Qo and k = log(F/100) / log(2)
The total cost, T, to produce a quantity Q units or lots can be obtained by integrating equation (2b) over the limits q = 0 to q = Q:
T = C1* q ^ kdq = C1*Q ^ (k+ 1)/(k+ 1) (3)
The average cost, a, per unit or lot quantity is the total cost divided by the quantity:
A = T/Q (4)
For processes where the experience factor is accurately known, the average cost is often used to quote a lot or piece price to be effective over the entire production. Suppose, for example, that a first lot of ten pieces is produced at a cost of $20.00 by a process with a known experience factor of 80%. What would be the predicted piece cost for 1,000 units? For F = 80%, k is found to be log (0.80)/log (2) = 20.3219, and for this case the “experience” quantity Q = 1,000/10 = 100.
Therefore:
C = 20.00*100 ^ (-0.3219) = 4.5412
So that at the end of the run the production cost has declined to $4.54 per lot. The total cost, from equation (3), becomes:
T = 20*100 ^ (0.6781)/0.6781 = 669.7274
The average production cost per unit quantity (1 lot) is therefore T/Q = $6.70 and the piece cost is about $0.67. This approach can be used to create log-log plots for various experience factors, giving unit costs as a function of quantities and initial costs. For example, a process with 80% experience factor and an initial cost of 1.00 per unit can expect unit costs to decline to about 0.11 by the time 1,024 (2 ^ 10) units have been produced. This not atypical of the semiconductor industry, where F may be 75% or even less. At the other end of the scale a complex, low volume product may be 90% or higher. One-offs with highly customized assemblies will be as high as 100%: the product lifetime is too short (one-off) and the standardized process component(s) are too limited to offer meaningful improvement opportunities.
New Technologies—the Experience Factor[1]
To use this analysis for new technologies it is necessary to determine the experience factor. This can done using a broader experience base than the simple doubling shown in equation (1) by flipping equation (2a) around [. . .] [LL1] provided the data are available, specifically:
F = 10 ^ (log(2)*log(C/C1) /log(Q)) (5)
If the production cost of a metal-core type insulated metal substrate LED multichip board was 2.00 when 10,000 pieces had been produced (C1) and the cost (C) is now 0.65 when 4,000,000 have been produced (Q = 400), what is the experience factor F?
F = 10 ^ (log(2)*log(0.65/2.00)/log(400))
Or: F = 0.878
What will be the cost for the 20,000,000th piece when Q will be effectively 2,000 (20,000,000/10,000)?
k = log(0:878)/log(2) = 20:18771
C = 2.00*2000 ^ (20.18771) = 0.4801
This example assumes a limited degree of process innovation is necessary in the introduction of a new layout for the same function/substrate. As is often the case in printed circuit manufacturing, where the emphasis is less on products and more on capabilities built on standardized processes, the experience factor may be even higher than 88%. It is important to remember that the experience factor “F” does not imply any particular degree of expertise or mastery of the technology. It is simply an index of the expected stability of processing costs over the lifetime of the design.
References
- Burr, W., Pearne, N., “Learning curve theory and innovation,” Circuit World, Vol. 39, Issue 4, 2003, pp 169–173.
- Transformative Learning (Jack Mezirow)
- www.wikipedia.org
- The Quintessential of Generative Learning Theory
- The Learning Curve or Experience Curve, provided by James Martin.
Happy Holden has worked in printed circuit technology since 1970 with Hewlett-Packard, NanYa/Westwood, Merix, Foxconn and Gentex. Currently, he is the co-editor, with Clyde Coombs, of the Printed Circuit Handbook, 7th Ed.
More Columns from Happy’s Tech Talk
Happy’s Tech Talk #40: Factors in PTH Reliability—Hole VoidsHappy’s Tech Talk #39: PCBs Replace Motor Windings
Happy’s Tech Talk #38: Novel Metallization for UHDI
Happy’s Tech Talk #37: New Ultra HDI Materials
Happy’s Tech Talk #36: The LEGO Principle of Optical Assembly
Happy’s Tech Talk #35: Yields March to Design Rules
Happy’s Tech Talk #34: Producibility and Other Pseudo-metrics
Happy’s Tech Talk #33: Wet Process Management and Control