Getting More Miles From Plug-in Hybrids
March 2, 2016 | University of California, RiversideEstimated reading time: 3 minutes
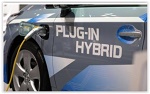
Plug-in hybrid electric vehicles (PHEVs) can reduce fuel consumption and greenhouse gas emissions compared to their gas-only counterparts. Researchers at the University of California, Riverside’s Bourns College of Engineeringhave taken the technology one step further, demonstrating how to improve the efficiency of current PHEVs by almost 12 percent.
Researchers at the University of California, Riverside have shown how to improve the efficiency of current PHEVs.
Since plug-in hybrids combine gas or diesel engines with electric motors and large rechargeable batteries, a key component is an energy management system (EMS) that controls when they switch from ‘all-electric’ mode, during which stored energy from their batteries is used, to ‘hybrid’ mode, which utilizes both fuel and electricity. As new EMS devices are developed, an important consideration is combining the power streams from both sources in the most energy-efficient way.
While not all plug-in hybrids work the same way, most start in all-electric mode, running on electricity until their battery pack is depleted and then switching to hybrid mode. Known as binary mode control, this EMS strategy is easy to apply, but isn’t the most efficient way to combine the two power sources. In lab tests, blended discharge strategies, in which power from the battery is used throughout the trip, have proven to be more efficient at minimizing fuel consumption and emissions, but until now they haven’t been a realistic option for real-world applications, said Xuewei Qi, a graduate student in the Bourns College of Engineering’s Center for Environmental Research and Technology (CE-CERT) who led the research. Qi is working with CE-CERT Director Matthew Barth, a professor of electrical and computer engineering.
“Blended discharge strategies have the ability to be extremely energy efficient, but those proposed previously require upfront knowledge about the nature of the trip, road conditions and traffic information, which in reality is almost impossible to provide,” Qi said.
While the UCR EMS does require trip-related information, it also gathers data in real time using onboard sensors and communications devices, rather than demanding it upfront. It is one of the first systems based on a machine learning technique called reinforcement learning (RL), and was published online Feb. 5 in the journal Transportation Research Record.
In comparison-based tests on a 20-mile commute in Southern California, the UCR EMS outperformed currently available binary mode systems, with average fuel savings of 11.9 percent. Even better, Qi said, the system gets smarter the more it’s used and is not model- or driver-specific, meaning it can be applied to any PHEV driven by any individual.
“In our reinforcement learning system, the vehicle learns everything it needs to be energy efficient based on historical data. As more data are gathered and evaluated, the system becomes better at making decisions that will save on energy,” Qi said.
Qi said the next phase of the research will focus on creating a cloud-based network that enables PHEVs to work together for even better results.
“Our current findings have shown how individual vehicles can learn from their historical driving behavior to operate in an energy efficient manner. The next step is to extend the proposed mode to a cloud-based vehicle network where vehicles not only learn from themselves but also each other. This will enable them to operate on even less fuel and will have a huge impact on the amount of greenhouse gases and other pollutants released,” he said.
The work was done by Qi and Barth, together with Guoyuan Wu, assistant research engineer at CE-CERT; Kanok Boriboonsomsin, associate research engineer at CE-CERT; and Jeffrey Gonder, senior engineer at the National Renewable Energy Laboratory in Golden, Colo. The project was partially supported by the U. S. Department of Transportation.
Suggested Items
Lockheed Martin Australia, The Department Of Defence Sign Strategic Partnership Head Contract
04/26/2024 | Lockheed MartinLockheed Martin Australia signed a landmark AUD$500 million contract with the Department of Defence to build Australia’s future Joint Air Battle Management System under project - AIR6500 Phase 1 (AIR6500-1).
Cadence, TSMC Collaborate on Wide-Ranging Innovations to Transform System and Semiconductor Design
04/25/2024 | Cadence Design SystemsCadence Design Systems, Inc. and TSMC have extended their longstanding collaboration by announcing a broad range of innovative technology advancements to accelerate design, including developments ranging from 3D-IC and advanced process nodes to design IP and photonics.
Siemens’ Breakthrough Veloce CS Transforms Emulation and Prototyping with Three Novel Products
04/24/2024 | Siemens Digital Industries SoftwareSiemens Digital Industries Software launched the Veloce™ CS hardware-assisted verification and validation system. In a first for the EDA (Electronic Design Automation) industry, Veloce CS incorporates hardware emulation, enterprise prototyping and software prototyping and is built on two highly advanced integrated circuits (ICs) – Siemens’ new, purpose-built Crystal accelerator chip for emulation and the AMD Versal™ Premium VP1902 FPGA adaptive SoC (System-on-a-chip) for enterprise and software prototyping.
Taiyo Circuit Automation Installs New DP3500 into Fuba Printed Circuits, Tunisia
04/25/2024 | Taiyo Circuit AutomationTaiyo Circuit Automation is proud to be partnered with Fuba Printed Circuits, Tunisia part of the OneTech Group of companies, a leading printed circuit board manufacturer based out of Bizerte, Tunisia, on their first installation of Taiyo Circuit Automation DP3500 coater.
Vicor Power Orders Hentec Industries/RPS Automation Pulsar Solderability Testing System
04/24/2024 | Hentec Industries/RPS AutomationHentec Industries/RPS Automation, a leading manufacturer of selective soldering, lead tinning and solderability test equipment, is pleased to announce that Vicor Power has finalized the purchase of a Pulsar solderability testing system.