Engineers Improve Electrochemical Sensing by Incorporating Machine Learning
November 28, 2022 | Pennsylvania State UniversityEstimated reading time: 3 minutes
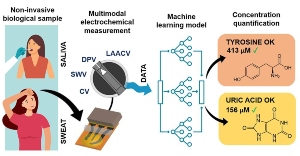
Combining machine learning with multimodal electrochemical sensing can significantly improve the analytical performance of biosensors, according to new findings from a Penn State research team. These improvements may benefit noninvasive health monitoring, such as testing that involves saliva or sweat. The findings were published this month in Analytica Chimica Acta.
The researchers developed a novel analytical platform that enabled them to selectively measure multiple biomolecules using a single sensor, saving space and reducing complexity as compared to the usual route of using multi-sensor systems. In particular, they showed that their sensor can simultaneously detect small quantities of uric acid and tyrosine — two important biomarkers associated with kidney and cardiovascular diseases, diabetes, metabolic disorders, and neuropsychiatric and eating disorders — in sweat and saliva, making the developed method suitable for personalized health monitoring and intervention.
Many biomarkers have similar molecular structures or overlapping electrochemical signatures, making it difficult to detect them simultaneously. Leveraging machine learning for measuring multiple biomarkers can improve the accuracy and reliability of diagnostics and as a result improve patient outcomes, according to the researchers. Further, sensing using the same device saves resources and biological sample volumes needed for tests, which is critical with clinical samples with scarce amounts.
“We developed a new approach to improve the performance of electrochemical biosensors by combining machine learning with multimodal measurement,” said Aida Ebrahimi, Thomas and Sheila Roell Early Career Assistant Professor of Electrical Engineering and assistant professor of biomedical engineering. “Using our optimized machine learning architecture, we could detect biomolecules in amounts 100 times lower than what conventional sensing methods can do.”
The researchers’ methodology features a hardware/software system that enables them to automatically gather and process information based on a machine learning model that is trained to identify biomolecules in biological fluids such as saliva and sweat, which are common choices for noninvasive health monitoring.
“The machine learning-powered electrochemical diagnostic approach presented in this paper may find broader application in multiplexed biochemical sensing,” said Vinay Kammarchedu, 2022-23 Milton and Albertha Langdon Memorial Graduate Fellow in Electrical Engineering at Penn State and first author on the paper. “For example, this method can be extended to a variety of other molecules, including food and water toxins, drugs and neurochemicals that are challenging to detect simultaneously using conventional electrochemical methods.”
In their ongoing work, the researchers are applying this approach on such neurochemicals, which are difficult to detect due to similarities in their molecular structure and overlapping electrochemical signatures.
“Our methodology successfully used one material to differentiate and distinguish four neurochemicals that are important in diseases like Parkinson’s and Alzheimer’s,” Ebrahimi said. “While this preliminary data is promising, we must work further to be able to detect the lower levels of these neurochemicals in biological samples such as saliva.”
Beyond the specific results with the uric acid and tyrosine, the researchers are excited about the potential and versatility of the methodology.
“It is a new way of designing electrochemical diagnostic methods that may be applied to a variety of applications beyond biomedical systems,” Ebrahimi said.
Combined with innovations in material and device engineering for sensor development, the researchers’ analytical method may provide opportunities in pharmaceuticals, life science research, food screening, detection of environmental toxins and biodefense, where accurate and multiplexed testing or in-line monitoring is needed.
Conventionally, multiplexing is achieved by spectroscopic methods that rely on bulky and expensive equipment that is more suited for lab-based analysis. In the researchers’ current prototype stage, the hardware is benchtop sized. They are working to make a smaller system that can be implemented for more than just health monitoring.
“Ultimately, we envision a handheld and field-deployable device that will be easier to use and more readily available than the current practices used in laboratory or clinical settings,” Kammarchedu said.
The research was funded by Phase II of the National Science Foundation (NSF) Industry-University Cooperative Research Centers Program (I/UCRC). Derrick Butler, who was a doctoral student of electrical engineering during this project, contributed to this research. Kammarchedu, Butler and Ebrahimi are also affiliated with the Center for Atomically Thin Multifunctional Coatings (ATOMIC), which is an NSF:I/UCRC Center in the Materials Research Institute at Penn State.
Suggested Items
Indium Corporation Expert to Present on Pb-Free Solder for Die-Attach in Discrete Power Applications
04/30/2024 | Indium CorporationIndium Corporation Product Manager – Semiconductor Dean Payne will present at the Advanced Packaging for Power Electronics conference, hosted by IMAPS, held May 8-9 in Woburn, Massachusetts, USA.
Boeing Opens Research & Technology Center in Japan
04/23/2024 | BoeingBoeing today opened a Boeing Research & Technology (BR&T) Center in Japan that will focus on innovation to enable the commercial aviation industry meet its goal of net zero carbon emissions by 2050.
Ansys Joins BAE Systems’ Mission Advantage Program to Advance Digital Engineering Across US Department of Defense
04/16/2024 | ANSYSAnsys announced it is working with BAE Systems, Inc., to accelerate the adoption of digital engineering and MBSE across the Department of Defense (DoD).
Incap Estonia Teamed Up With Solaride to Fuel Youth Excitement for The World of Engineering
04/11/2024 | IncapIncap Estonia partnered with Solaride to ignite enthusiasm among young people for the world of engineering and sustainable technology. Together, they organised an inspiring day for students from grades 7 through 12 in Saaremaa. Held at Incap’s Kuressaare factory, this event brought together over 130 young minds eager to explore the innovative world of technology and learn more about advanced electronics manufacturing.
Mark Schulman Rocks Zuken Innovation World + integrate24
04/10/2024 | ZukenZuken USA, Inc. is excited to announce that the renowned drummer and motivational speaker, Mark Schulman, will be the keynote speaker at this year's Zuken Innovation World Americas (ZIW) conference, co-located with integrate24. Scheduled for September 17-19, 2024, in the vibrant city of Cleveland, Ohio, the conference aims to bring together the brightest minds in the PCB, Wire Harness, and Digital Engineering fields.