Machine Learning Techniques Improve X-ray Materials Analysis
November 21, 2023 | ACN NewswireEstimated reading time: 2 minutes
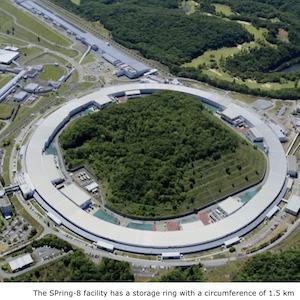
Researchers of RIKEN at Japan’s state-of-the-art synchrotron radiation facility, SPring-8, and their collaborators, have developed a faster and simpler way to carry out segmentation analysis, a vital process in materials science. The new method was published in the journal Science and Technology of Advanced Materials: Methods.
Segmentation analysis is used to understand the fine-scale composition of a material. It identifies distinct regions (or ‘segments’) with specific compositions, structural characteristics, or properties. This helps evaluate the suitability of a material for specific functions, as well as its possible limitations. It can also be used for quality control in material fabrication and for identifying points of weakness when analyzing materials that have failed.
Segmentation analysis is very important for synchrotron radiation X-ray computed tomography (SR-CT), which is similar to conventional medical CT scanning but uses intense focused X-rays produced by electrons circulating in a storage ring at nearly the speed of light. The team have demonstrated that machine learning is capable in conducting the segmentation analysis for the refraction contrast CT, which is especially useful for visualizing the three-dimensional structure in samples with small density differences between regions of interest, such as epoxy resins.
“Until now, no general segmentation analysis method for synchrotron radiation refraction contrast CT has been reported,” says first author Satoru Hamamoto. “Researchers have generally had to do segmentation analysis by trial and error, which has made it difficult for those who are not experts.”
The team’s solution was to use machine learning methods established in biomedical fields in combination with a transfer learning technique to finely adjust to the segmentation analysis of SR-CTs. Building on the existing machine learning model greatly reduced the amount of training data needed to get results.
“We’ve demonstrated that fast and accurate segmentation analysis is possible using machine learning methods, at a reasonable computational cost, and in a way that should allow non-experts to achieve levels of accuracy similar to experts,” says Takaki Hatsui, who led the research group.
The researchers carried out a proof-of-concept analysis in which they successfully detected regions created by water within an epoxy resin. Their success suggests that the technique will be useful for analyzing a wide range of materials.
To make this analysis method available as widely and quickly as possible, the team plans to establish segmentation analysis as a service offered to external researchers by the SPring-8 data center, which has recently started its operation.
Suggested Items
DARPA Taps RTX to Strengthen Cyber Resiliency
11/11/2024 | RTXRTX's BBN Technologies was awarded a contract to support DARPA's Compartmentalization and Privilege Management, or CPM, program. The CPM program aims to enhance cyber resilience by automatically subdividing software systems into smaller, secure compartments, preventing initial breaches from escalating into successful cyberattacks while maintaining system efficiency.
The Quest for Perfect Products
11/11/2024 | Marcy LaRont, I-Connect007Anna-Katrina Shedletsky is a former Apple engineer who formed her own company, Instrumental, to address what she felt were frustrating and costly engineering problems and inefficiencies at Apple. She’s passionate about her quest to build perfect products faster than ever before. In this interview, Anna shares her journey from creating innovative products like the Apple Watch to addressing the complexities of failure analysis in manufacturing. She also discusses an upcoming educational webinar she is hosting with Valentina Ratner, CEO of AllSpice.io, for engineering leaders in the manufacturing space. They look forward to providing “real talk” and value to their engineering colleagues.
TSMC Recognizes Ansys for Excellence in Design Enablement for AI, HPC, and Photonics Silicon Systems
10/28/2024 | ANSYSAnsys was recognized at the TSMC 2024 Open Innovation Platform® (OIP) Partner of the Year awards for excellence in design enablement for AI, HPC, and photonics silicon systems.
CACI Awarded $805 Million Task Order to Provide Engineering Support and Technology to U.S. Navy’s NavalX Office
10/22/2024 | CACI International Inc.CACI International Inc announced that it has been awarded a five-year task order valued at up to $805 million to provide engineering support and technology to the U.S. Navy’s NavalX Office under the Department of Defense Information Analysis Center’s (DoD IAC) multiple-award contract (MAC) vehicle.
SEMI Energy Collaborative Releases Recommendations for Expanding Low Carbon Energy in Taiwan
10/16/2024 | SEMISEMI, the global industry association representing the semiconductor and electronics design and manufacturing supply chain, released a report by its Energy Collaborative (EC) with recommendations on expanding low-carbon energy (LCE) availability in Taiwan.