-
- News
- Books
Featured Books
- pcb007 Magazine
Latest Issues
Current IssueVoices of the Industry
We take the pulse of the PCB industry by sharing insights from leading fabricators and suppliers in this month's issue. We've gathered their thoughts on the new U.S. administration, spending, the war in Ukraine, and their most pressing needs. It’s an eye-opening and enlightening look behind the curtain.
The Essential Guide to Surface Finishes
We go back to basics this month with a recount of a little history, and look forward to addressing the many challenges that high density, high frequency, adhesion, SI, and corrosion concerns for harsh environments bring to the fore. We compare and contrast surface finishes by type and application, take a hard look at the many iterations of gold plating, and address palladium as a surface finish.
It's Show Time!
In this month’s issue of PCB007 Magazine we reimagine the possibilities featuring stories all about IPC APEX EXPO 2025—covering what to look forward to, and what you don’t want to miss.
- Articles
- Columns
Search Console
- Links
- Media kit
||| MENU - pcb007 Magazine
Siemens Simplifies Development of AI Accelerators for Advanced System-on-chip Designs with Catapult AI NN
May 24, 2024 | SiemensEstimated reading time: 2 minutes
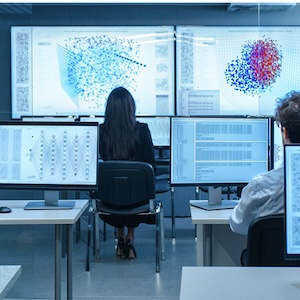
Siemens Digital Industries Software announced Catapult™ AI NN software for High-Level Synthesis (HLS) of neural network accelerators on Application-Specific Integrated Circuits (ASICs) and System-on-a-chip (SoCs). Catapult AI NN is a complete solution that starts with a neural network description from an AI framework, converts it into C++ and synthesizes it into an RTL accelerator in Verilog or VHDL for implementation in silicon.
Catapult AI NN brings together hls4ml, an open-source package for machine learning hardware acceleration, and Siemens' Catapult™ HLS software for High-Level Synthesis. Developed in close collaboration with Fermilab, a U.S. Department of Energy Laboratory, and other leading contributors to hls4ml, Catapult AI NN addresses the unique requirements of machine learning accelerator design for power, performance, and area on custom silicon.
“The handoff process and manual conversion of a neural network model into a hardware implementation is very inefficient, time consuming and error-prone, especially when it comes to creating and verifying variants of a hardware accelerator tailored to specific performance, power and area,” said Mo Movahed, Vice President and General Manager for High-Level Design, Verification and Power, Siemens Digital Industries Software. “By empowering scientists and AI experts to leverage industry-standard AI frameworks, such as neural network model design, and by seamlessly synthesizing these models into hardware designs optimized for power, performance, and area (PPA), we're opening a whole new realm of possibilities for AI and machine learning software engineers. Our new Catapult AI NN solution allows developers to automate and implement their neural network models for optimal PPA concurrently during the software development process, ushering in a new era of efficiency and innovation in AI development.”
As runtime AI and machine learning tasks migrate from the datacenter into everything from consumer appliances to medical devices, there is a rapidly growing requirement for "right-sized" AI hardware to minimize power consumption, lower cost and maximize end-product differentiation. However, most machine learning experts are more comfortable working with tools such as TensorFlow, PyTorch or Keras, rather than synthesizable C++, Verilog or VHDL. There has traditionally been no easy path for AI experts to accelerate their machine learning applications in a right-sized ASIC or SoC implementation.
The hls4ml initiative is intended to help bridge this gap by generating C++ from a neural network described in AI frameworks such as TensorFlow, PyTorch or Keras. The C++ can then be deployed for an FPGA, ASIC or SoC implementation.
Catapult AI NN extends the capabilities of hls4ml to ASIC and SoC design. It includes a dedicated library of specialized C++ machine learning functions that are tailored to ASIC design. Using these functions, designers can optimize PPA by making latency and resource trade-offs across alternative implementations from the C++ code. Moreover, designers can now evaluate the impact of different neural net designs to determine the best neural network structure for hardware.
"Particle detector applications have extremely stringent edge AI constraints," said Panagiotis Spentzouris, Fermilab Associate Lab Director for Emerging Technologies. “Through our collaboration with Siemens, we were able to develop Catapult AI NN, a synthesis framework that leverages the expertise of our scientists and AI experts without requiring them to become ASIC designers. Moreover, this powerful new framework is also ideal for seasoned hardware experts.”
Suggested Items
Ceva Neural Processing Unit IP for Edge AI Selected by Nextchip for Next-Generation ADAS Solutions
04/23/2025 | PRNewswireCeva, Inc., the leading licensor of silicon and software IP that enables Smart Edge devices to connect, sense and infer data more reliably and efficiently, announced that Nextchip has licensed the NeuPro-M Edge AI Neural Processing Unit (NPU) IP for its next-generation advanced driver assistance systems (ADAS) solutions.
UHDI Fundamentals: UHDI Advances Neurotechnology
03/05/2025 | Anaya Vardya, American Standard CircuitsUltra high density interconnect (UHDI) technology is revolutionizing the field of neurotechnology and brain-computer interfaces (BCIs) by enabling unprecedented levels of miniaturization, complexity, and performance in neural devices. Here’s an in-depth look at how UHDI contributes to these domains.
Pusan National University Develops One-Step 3D Microelectrode Technology for Neural Interfaces
02/28/2025 | PRNewswireNeural interfaces are crucial in restoring and enhancing impaired neural functions, but current technologies struggle to achieve close contact with soft and curved neural tissues. Researchers at Pusan National University have introduced an innovative method—microelectrothermoforming (μETF)—to create flexible neural interfaces with microscopic three-dimensional (3D) structures.
Delvitech Expands Into Microelectronics and Chiplet Segments
01/23/2025 | DelvitechDelvitech, a global leader in AI-driven optical inspection technology, is proud to announce its strategic expansion into the microelectronics and chiplet sectors.
STMicroelectronics to Boost AI at the Edge with New NPU-Accelerated STM32 Microcontrollers
12/11/2024 | STMicroelectronicsSTMicroelectronics, is making embedded artificial intelligence (AI) truly here-to-help with a new microcontroller series integrating, for the first time, accelerated machine-learning (ML) capabilities.