-
- News
- Books
Featured Books
- smt007 Magazine
Latest Issues
Current IssueWhat's Your Sweet Spot?
Are you in a niche that’s growing or shrinking? Is it time to reassess and refocus? We spotlight companies thriving by redefining or reinforcing their niche. What are their insights?
Moving Forward With Confidence
In this issue, we focus on sales and quoting, workforce training, new IPC leadership in the U.S. and Canada, the effects of tariffs, CFX standards, and much more—all designed to provide perspective as you move through the cloud bank of today's shifting economic market.
Intelligent Test and Inspection
Are you ready to explore the cutting-edge advancements shaping the electronics manufacturing industry? The May 2025 issue of SMT007 Magazine is packed with insights, innovations, and expert perspectives that you won’t want to miss.
- Articles
- Columns
- Links
- Media kit
||| MENU - smt007 Magazine
Estimated reading time: 6 minutes
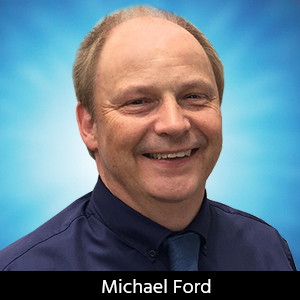
Smart Factory Insights: It’s Not What You Have—It’s How You Use It
According to the reports, all the machines in the factory are performing well, but the factory itself appears to be in a coma, unable to fulfill critical delivery requirements. Is this a nightmare scenario, or is it happening every day? Trying to help, some managers are requesting further investment in automation, while others are demanding better machine data that explains where it all went wrong. Digital technology to the rescue, or is it making the problem worse?
Having machine data—or not having it—is not an indication of good or bad. My evil self could go into any factory shut down due to COVID-19 today and find ways to take out production reports that show that over the recent period, no scrap was made, no parts were lost, there were no line imbalances, the factory achieved zero-defects, there were no missed deliveries, and there was no sign of productivity loss on any machine. No time was lost for manual operators taking restroom breaks, there were no accidents, nobody was late for work, and there was no need for any overtime. In different circumstances, these statistics would be excellent news, and while they may be true, they don’t have any meaning. These statistics would show that you can take a certain view with data and make a case to justify all manner of things.
Though perhaps not as extreme, this practice is happening all the time. Metrics are crafted from simple data sources to promote the positive; after all, we each look forward to a good review at the end of the year, but isolated “facts” can hide an overall negative situation. Money first seeps, then pours through the cracks, covering over fundamental operational issues for which there is little or no visibility other than symptoms that appear as business limitations or even failure.
Analysis of data needs to be more intelligent. Machine learning and line-based closed-loop systems are great at using raw machine data to automate process improvement. Beyond these narrow solutions, however, the analysis of machine data in isolation is relatively pointless. We would expect these days that automated machines work well when able to do so, providing that positive spin opportunity. The real challenge is how to perform analyses of what is happening in between the machines, where there is no data being reported.
At the simple level, no machine data means potential loss. Machines are stopped and perhaps blocked, starved, not needed, or broken down; there could be material issues, quality concerns, lack of operators, a scheduled vacation, or even a pandemic. The machines don’t know; they only say that they are stopped. Through the correlation of data from multiple disciplines—such as material logistics, planning, and quality management—what needs to be discovered are the root causes and net effects of any key exception in the process preventing operational progress.
Then, there is a more complex level. We should look at the progress of a product through manufacturing rather than just looking at the performance of the machines themselves. Consider the typical international tourist experience at an airport. How much time is actually needed to check-in, drop a bag, enjoy the security check, walk to the gate, and get on the plane? Probably about 10 minutes door to door, but we are told to arrive at the airport at least two hours before the flight leaves. Therefore, added-value time at the airport is about 8%, and the other 92% is waste, but owners of the shops and cafés may tend to disagree.
To report about 8% efficiency in manufacturing would probably get you fired, but I could go into most factories working normally today and get reports that show efficiencies measured in such a way as being much worse than 8%. We are fixated by looking at machine data rather than using the data to truly analyze the effectiveness of the factory in doing its job, taking materials, and making end products. The stock of raw materials should be minimized, as should be the holding of sub-assemblies, areas of semi-finished goods, and finished goods in the warehouse. We should not have so many products awaiting repair or retest, being repaired or tested, going through quality inspection, being in quarantine, or being piled up in front of processes that are not yet set up and ready to execute. All of these aspects of manufacturing have a far more significant effect on the business than the simple operation of any particular machine.
Machine data acquisition has been revolutionized of late, with data gathering from machines being easier, more detailed, timely, and accurate without the need for middleware or customized machine interfaces. This is notably true in the case of using the IPC Connected Factory Exchange (CFX) standard.
There are no interfaces for the gaps in between the machines. These are the areas that have a major impact on the operation. Take the example of an individual product simply leaving one process and moving to another. The product gets to the end of the line and stops. It is stored—somewhere, somehow—waiting for the others in the batch, job, or work order to be completed. The next process has to be as efficient as possible, so planning delayed the start time until it was sure that all products had been completed by the prior process, a vacancy had opened up, and it was optimal timing to do so.
Minutes, hours, or days could pass. There’s so much increased opportunity for handling issues, further delays from failing equipment, missing materials, effects of engineering revisions, and contamination, resulting potentially in more inspection, cleaning, processing, and more delays and storage. Being able to digitally track the paths of products during assembly creates significantly more opportunities for efficiency improvement and cost savings. To do this, the data from individual machines and processes also needs to be used to create a live virtual “movie” of everything happening in the factory—a live manufacturing “digital twin” that’s the real thing, not a simulation.
Unlike people, software does not need to create fancy 3D animations and images to be able to apply a rules-based engine that takes contextualization of data holistically from machines, materials, quality, and planning, as well as knowledge of working line configurations and products to create an omniperspective-based digital twin model. The manufacturing digital twin “movie” extends back in time in terms of near-term performance history to learn what works well and where everything currently is. It also extends forward in time, as extrapolations based on current trends are analyzed, to detect any issues that could be avoided by implementing changes and decisions now.
In effect, this rules-based manufacturing digital twin is controlling and managing the whole production operation, creating visibility and automation around challenges and addressing the core business needs and improvement opportunities within the factory. This is no ordinary MES solution; instead, it is the redefined, modern IIoT-driven MES solution built specifically around the rules-based digital twin architecture.
Therefore, gathering data from around the factory is step one toward making digital solutions work for the betterment of manufacturing. However, you do need to take more than one step to get to the next level. How you use the data is far more significant than just having it, making dashboards from it, and performing machine learning and analytics. The true digital twin for manufacturing within IIoT-based MES execution is here.
This column originally appeared in the June issue of SMT007 Magazine.
More Columns from Smart Factory Insights
Smart Factory Insights: Making Rework a Smart Business OpportunitySmart Factory Insights: The Sustainability Gold Rush
Smart Factory Insights: Today’s Manufacturing Jobs Require a New Skill Set
Smart Factory Insights: Compose Yourself, Mr. Ford
Smart Factory Insights: The Smart Business Case for Local PCB Manufacturing
Smart Factory Insights: Manufacturing Digital Twin—Spanners in the Works
Smart Factory Insights: Machines, People, and AI
Smart Factory Insights: Is Sustainability in Manufacturing a Benefit or Burden?