-
- News
- Books
Featured Books
- smt007 Magazine
Latest Issues
Current IssueDo You Have X-ray Vision?
Has X-ray’s time finally come in electronics manufacturing? Join us in this issue of SMT007 Magazine, where we answer this question and others to bring more efficiency to your bottom line.
IPC APEX EXPO 2025: A Preview
It’s that time again. If you’re going to Anaheim for IPC APEX EXPO 2025, we’ll see you there. In the meantime, consider this issue of SMT007 Magazine to be your golden ticket to planning the show.
Technical Resources
Key industry organizations–all with knowledge sharing as a part of their mission–share their technical repositories in this issue of SMT007 Magazine. Where can you find information critical to your work? Odds are, right here.
- Articles
- Columns
Search Console
- Links
- Media kit
||| MENU - smt007 Magazine
Estimated reading time: 8 minutes
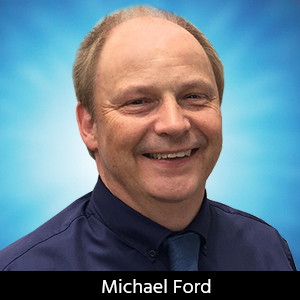
Smart Factory Insights: Smart Factories—Indirectly the Death of Test and Inspection
The indirect role played by test and inspection has no place in the smart factory if it is there simply to detect defects, providing a filter, at best, and acting for the improvement of product reliability in the market—a response to mistakes and weaknesses within manufacturing, effectively, or a tax on inadequacy. In the smart factory, test and inspection are reinvented, contributing direct added value, playing a new and critically important role where defects are avoided through the use of data and create a completely different value proposition. How can the digitalized Deming Theory be explained to those managing budgets and investments to ensure that we move our operations forward digitally in the best way possible?
Going back to basics, the concept of “direct” vs. “indirect” value to manufacturing is a fundamental measurement of the core business performance of any manufacturing operation. The value of the metric is the measurement of efficiency of any expense or cost related to manufacturing support, including non-added-value processes, in comparison to resources that are performing added-value manufacturing. Most other metrics focus on breaking down production activities, allowing inefficiencies in support, and enabling activities to go unchallenged. These include the operational costs of test and inspection, as well as physical activities orchestrated, managed, and monitored by MES, such as supply-chain logistics and quality management.
The origin of this metric predates automation and is associated with the work of W. Edwards Deming, though his dream had to be fulfilled with armies of staff, paperwork, and processes. Now, we have digitized it. As production operators represent the major operational fixed cost, those who perform assembly are seen as direct added-value, whereas other activities—including repair and re-work—are seen as being indirect.
Applying the same principle to test and inspection, imagine a set of production processes where there is one machine, such as a pick-and-place, and a reflow oven both involved in the making of the product, and then an SPI, AOI, and ICT machine. Applying the direct/indirect rule, the direct ratio of this line is just 40%. In a perfect world, we would only need the two direct machines making the product. It means that considerable investment has been made, together with a high proportion of the operational cost, which is—in a sense—waste.
However, few would be bold enough to do without the test and inspection machines, as quality performance would no doubt suffer, bringing potentially more substantial costs associated with poor quality later. The reluctance to invest in equipment and software solutions that are regarded as being indirect is understandable. But as this and other similar business-level metrics are adversely affected, this leads to concerns with business sponsors.
How poor is the actual team at the site that they need so much more indirect support than others? Why should we invest in their inadequacy? These are old-school questions that the smart factory still has to answer if investment in smart technology associated with test and inspection, as well as software solutions, are to be readily accepted, as opposed to being a continuous battle for funding.
The fact that the smart factory is run on the contextualization of data provided by the IIoT-driven MES solution’s built-in defined ontology is very fortunate for vendors of test and inspection equipment. These processes actually provide a large part of the required data for smart decision-making. Can we finally put aside the stigma that these machines and solutions are indirect costs, and position them as adding direct value to the production operation? We need to bear in mind that the key people we need to convince are not technically minded. The engineering-orientated explanation of how the role of these machines has changed is not going to be easy.
Let’s first take a look at where we are in terms of maturity with the use of test and inspection data, as the technology is not yet at the point where it can be regarded as mature. Many years ago, the application of Six Sigma showed that defect occurrence is not required and is avoided by understanding the variations in processes and how to control them. Analysis of the data statistically as machine learning, closed-loop solutions, or as MES-level quality analysis exposes trends that risk defects, communicating the issue—and even executing the appropriate corrective action—before any defect occurs.
Whilst this is simple in theory, the practice itself is multi-dimensional, as there are so many complex and interacting factors. Machine-learning and closed-loop algorithms are yet to be perfected to the point where the root cause of variation can be correctly identified and appropriate action taken. A simple example of this is the analysis of the deviation of placement coordinates, comparing positions as specified in the machine program against those inspected after placement.
Simply put, too much deviation raises the alarm before the risk of making a poor joint or an unwanted short. However, how much is too much depends on many factors, such as the size, shape, and orientation of the pads on the PCB. Consider the size, shape, pitch, and contact profile of component leads, as well as the location, size, shape, and thickness of the solder paste.
Then, there is the need to understand the nature of the patterns of deviation, especially with respect to adjacent parts. Patterns in the data may suggest a PCB condition—for example, stretch or twist, which is an issue around a specific nozzle that has been used—or be related to the specific part itself. Each of these factors comes with its own set of rules as to what can or cannot be tolerated, as well as what potential adjustments in any preceding processes may be applied.
In reality, all of these effects happen concurrently with different levels of contribution. With all the analysis done, that is not the end of it, as certain aspects of trend calculations need to be reset when, for example, PCB packs change, nozzles are cleaned or replaced, materials replenished, machine parameters adjusted, etc. A truly challenging AI application running within a truly big data environment, with data from design, the supply chain, machine programs, MES, and other sources all needed to provide a holistic machine-learning or closed-loop solution. And this was a “simple” example. It is not quite a done deal as to whether we can say that “defects are history” already, though a lot of people are working hard on these algorithms from many directions, so we get closer and closer.
Business-wise, to get the buy-in to invest in smart factory inspection and test machines—as well as IIoT-based MES solutions—we need to create a convincing argument that shows that they are an essential and intrinsic part of the value-added process. Direct machines today already include operations that are not strictly of direct added value. Reading fiducial locations on the PCB increases the accuracy of placement, as does the taking of the picked-up component to a camera for recognition and alignment before placement. A significant amount of machine run-time can be dedicated to these tasks, which are in addition to the basic pickup and placement.
As a whole, the industry sees these functions as being essential to the SMT placement operation, and that it is only through the use of these technologies that the machines have become capable of placing the newer, smaller, or higher pitch components successfully. The addition of post-placement inspection and test could be positioned as being an evolution of this. The only difference is that it takes a separate machine to perform the test and inspection, as well as external software for the machine-learning, line-learning closed-loop, or factory-learning at the MES level. However, they are all essentially the same in that they facilitate increased direct performance of the line itself.
As we have seen with the state of the technology as it evolves, the transition of test and inspection to become a zero-defect driver as opposed to being a filter of defects may not yet be black and white. For some time, there will be an element of both happening. As line and factory layers evolve, the expectation is that defects will be reduced and eliminated over time, as the data captured from test and inspection is improved, linked with increasing sources of data—such as that from MES—and algorithms at the machine.
The process has to start somewhere, though. Smart factory management must invest in initiatives that strive to make the transition from defect-based quality management to zero-defect quality management through the use of test and inspection data. I have seen yield losses reduce by an order of magnitude already through the use of software that utilizes test and inspection data to improve placement accuracies—a benefit that simply cannot be ignored.
Test, inspection, and IIoT-based MES software that support machine, line, and factory-learning belong in the must column of any smart factory shopping budget, justified as being of direct contribution to manufacturing, with an expectation that actual defect rates become very close to zero over a reasonable period.
If we are to truly embrace the potential of smart factories, we need to be able to utilize the latest revolutionary steps forward in technology that the industry has seen with standards-driven IIoT data exchange in the form of CFX, for instance, which virtually eliminate the costs of data acquisition. The use of IIoT-based machine-learning, closed-loop line learning—as well as contextualization of big data at the factory layer through the use of IIoT-driven MES solutions based on built-in ontology—are all things that are available now.
Significant benefits are already achievable, with the risk of non-added value investment eliminated—for example, in middleware and locked proprietary solutions. It is altogether great support for a manufacturing business strategy for success as we come to terms with increased global challenges, promoting flexible, sustainable, environmentally responsible, and profitable local manufacturing.
Michael Ford is the senior director of emerging industry strategy for Aegis Software.
This column originally appeared in the November 2020 issue of SMT007 Magazine.
More Columns from Smart Factory Insights
Smart Factory Insights: Making Rework a Smart Business OpportunitySmart Factory Insights: The Sustainability Gold Rush
Smart Factory Insights: Today’s Manufacturing Jobs Require a New Skill Set
Smart Factory Insights: Compose Yourself, Mr. Ford
Smart Factory Insights: The Smart Business Case for Local PCB Manufacturing
Smart Factory Insights: Manufacturing Digital Twin—Spanners in the Works
Smart Factory Insights: Machines, People, and AI
Smart Factory Insights: Is Sustainability in Manufacturing a Benefit or Burden?