-
- News
- Books
Featured Books
- smt007 Magazine
Latest Issues
Current IssueComing to Terms With AI
In this issue, we examine the profound effect artificial intelligence and machine learning are having on manufacturing and business processes. We follow technology, innovation, and money as automation becomes the new key indicator of growth in our industry.
Box Build
One trend is to add box build and final assembly to your product offering. In this issue, we explore the opportunities and risks of adding system assembly to your service portfolio.
IPC APEX EXPO 2024 Pre-show
This month’s issue devotes its pages to a comprehensive preview of the IPC APEX EXPO 2024 event. Whether your role is technical or business, if you're new-to-the-industry or seasoned veteran, you'll find value throughout this program.
- Articles
- Columns
Search Console
- Links
- Events
||| MENU - smt007 Magazine
Estimated reading time: 4 minutes
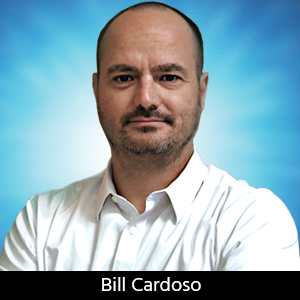
X-rayted Files: X-ray and AI—A Match Made In Heaven, Part 2
In Part 1 of this column series, I covered the basics of the relationship between X-ray inspection and artificial intelligence (AI). I’m now going to take a step forward to cover some of the practical ways we use AI to improve the efficiency of our X-ray inspections.
AI works by a learning process. The algorithms are fed a number of variables and outcomes associated with these variables. With enough inputs, the system will learn the correlating factors and then be able to predict the outcomes based on the input. For example, to create a model that classifies X-ray images as “pass” or “fail,” a number of pre-designated images must be uploaded into an image database. The software algorithms will then learn the features of the images that designate it “pass” or “fail.” As larger quantities of images are fed into the learning database, the confidence in the resulting prediction will increase.
One of the issues with automated X-ray inspection (AXI) is that it is limited in the natural variability that may occur. Perhaps it can be trained to only inspect solder voiding on BGAs, but it will not flag debris in a product. With AI, we can now account for known and unknown failure modes. Also, reliability is often placed on the operator of the machine to make a pass/fail judgment call. Operators are subject changing personnel, level of experience, and the human condition. Machine learning can perform these functions at incredible rates and is not subject to exhaustion.
Figure 1 shows a real case study performed on mobile phones. A large number of images classified as “good” and “bad” were fed into a database. This database of images was used to create a model that would analyze and classify new images. This AI model correctly located and flagged missing screws on the PCB inside of the phone.
Figure 1: Using AI to locate missing components.
The next study, as seen in the X-ray images in Figure 2, was a medical device packaging application. The device had to be packed in such a way that it could not be subjected to additional strain, weakening the structure of the tubing inside. The AI model picked up kinks in the tubing and flagged the location.
Figure 2: Using AI to identify product failures.
Another example of the use of AI to improve X-ray inspection is in the detection of counterfeit components. In this case, we introduced the use of the X-ray image as the unique fingerprint for an electronic component or printed circuit board assembly (PCBA). Solder voids, cracks, part alignment, die-attach porosity and voiding, die placement and alignment, and wire-bonding diagrams are just a few of the many unique features in the X-ray image that can be used in tandem to create a unique fingerprint for a single component or an entire PCBA. This technique can also be expanded to mechanical objects by utilizing other idiosyncratic features of the part, such as voids and porosity, to generate the X-ray image fingerprint.
To compare the acquired image with the original fingerprint, we study the use of AI as a powerful image comparison engine. The X-ray image fingerprint is calculated using unique algorithms and inserted into a custom database. Unlike taggants, the X-ray image technique does not allow for any adulteration because we are not adding any material to the component. Instead, the X-ray image fingerprint technique uses features of the material itself to generate the fingerprint.
Later in the supply chain, to read back these features to verify the authenticity of the component or PCBA, the user needs to image the part back with a compatible X-ray machine. The identification of the part will determine which locations and features are to be used to retrieve the fingerprint from the database. The same algorithms are then used to determine if any changes have occurred to the part and if the part is the same as introduced in the database.
It is important to note that much like taggants, the normal usage of the component or PCBAs—even under thermal cycling, humidity, and altitude variations—will not change the features utilized to create the X-ray image fingerprint of the parts. However, reworking these parts will cause a fingerprint mismatch. This is an added advantage of the X-ray image technique over taggants because—unlike taggants—X-ray image fingerprint can identify illicit reworked parts.
Figure 3 is an example of the X-ray image of a BGA assembly. This image shows the location of voids and porosity in the solder joints, which—when encoded—can be used to authenticate the sample. When determining the authenticity of electronic components, the X-ray fingerprint technique can use the wire bonds of the assembly.
Figure 4 shows an example of an electronic component, where the wire bonds are utilized as unique features to identify the sample.
We are constantly finding new applications for X-ray inspection and AI. As new machine-learning algorithms are developed, the integration of these techniques will continue to help us in the design of fully autonomous inspection systems.
Dr. Bill Cardoso is CEO of Creative Electron.
More Columns from X-Rayted Files
X-Rayted Files: Best Practices in Buying MachinesX-Rayted Files: ’23 and Me?
X-Rayted Files: Battery-Powered Advancements Keep on Ticking
X-Rayted Files: What’s Cooler Than X-ray?
X-Rayted Files: My Favorite BGA Assembly Ever, Part 3
X-Rayted Files: My Favorite BGA Assembly Ever, Part 2
X-Rayted Files: My Favorite BGA Assembly Ever
X-Rayted Files: Smart vs. Intelligent SMT Factory